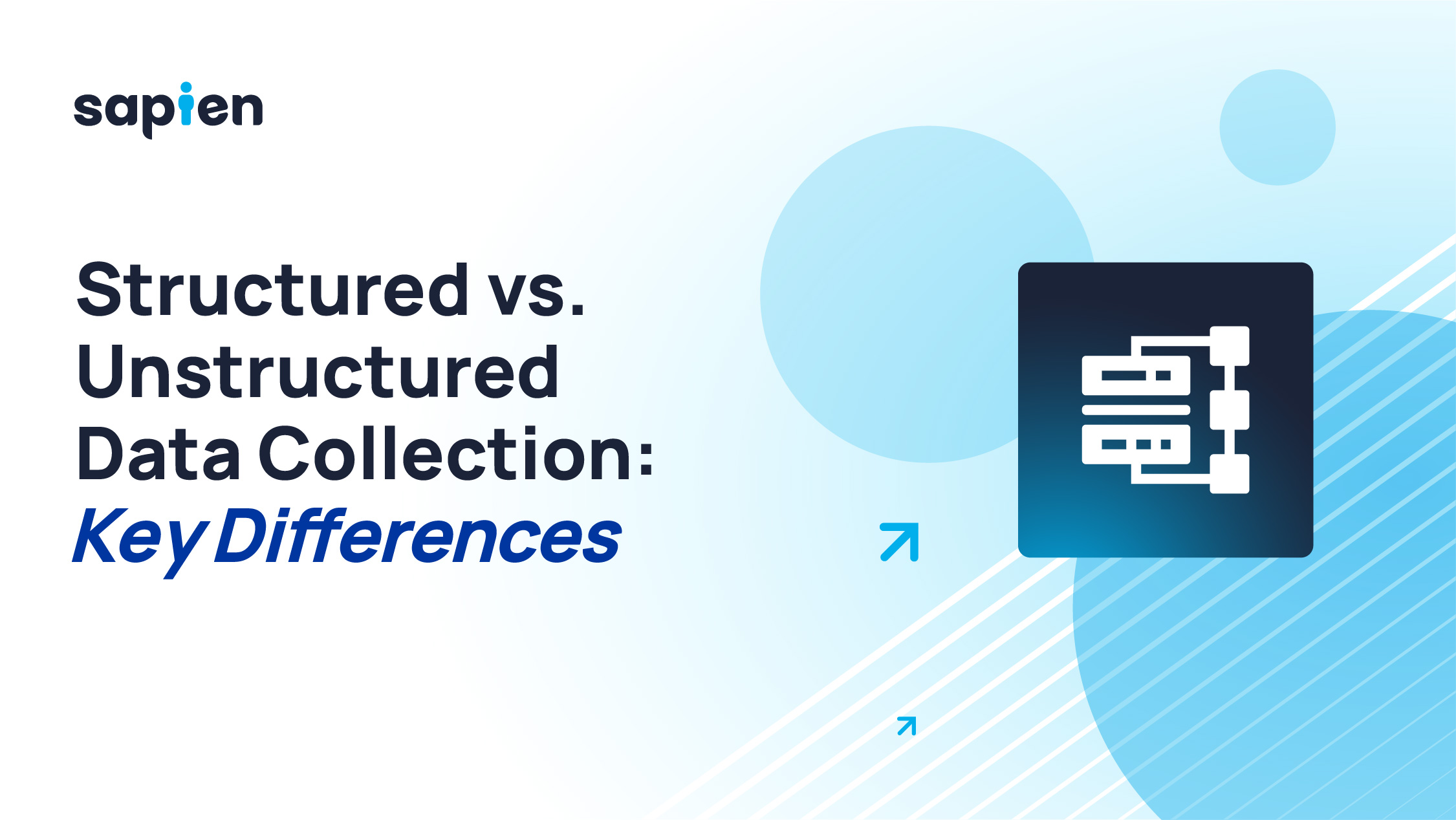
Data collection is a cornerstone of modern businesses, driving decisions, strategies, and innovations. In an era dominated by vast amounts of information, understanding how to collect, store, and utilize data is crucial for gaining a competitive edge. Among the different types of data collected, structured vs unstructured data are two of the most significant categories. Each serves a distinct purpose and comes with its own set of advantages and challenges.
This article will explore the key differences between structured and unstructured data, their use cases, and the essential factors businesses must consider when deciding how to collect and analyze each type of data.
Key Takeaways
- Choosing the Right Data Type: Understand the differences between structured and unstructured data to choose the best approach for your needs.
- Structured vs. Unstructured Data: Structured data is organized and easy to analyze, while unstructured data requires advanced tools like AI and machine learning.
- Advantages and Limitations: Structured data is simpler to process, while unstructured data offers more complex insights but requires more resources.
- Hybrid Approach: Combining structured and unstructured data provides a more comprehensive data analysis strategy.
Define Structured Data
Structured data refers to information that is highly organized and stored in a fixed format, typically in rows and columns within databases. It is often numeric or categorical and is easily accessible for querying and analysis. The structure makes it possible to utilize traditional data processing tools like SQL, which can efficiently manipulate and retrieve the data.
Structured data is commonly found in text datasets where the data points are well-defined, such as names, dates, or transaction details, making it straightforward to input, manage, and analyze using standard database systems. This consistency in format enables organizations to quickly extract insights and make data-driven decisions.
Key Features of Structured Data
- Format: Tables with rows and columns (e.g., SQL databases).
- Examples: Customer information (name, address, phone number), sales data, inventory records.
- Storage: Relational databases (RDBMS) like MySQL, PostgreSQL, or Oracle.
Practical Use Cases
Structured data is ideal for tasks requiring quick, accurate queries. Common use cases include:
- Customer Relationship Management (CRM): Storing customer profiles, purchase history, and interactions.
- Financial Transactions: Managing data related to banking, trading, and financial reporting.
- Inventory Management: Tracking products, stock levels, and pricing in retail businesses.
Define Unstructured Data
Unstructured data refers to any information that does not follow a predefined format or structure. It can include free text, images, audio, video, and other formats that are harder to categorize. This type of data requires more advanced processing techniques, including natural language processing (NLP) and machine learning (ML), to extract meaningful insights.
According to Forbes’s report, 80% of the world’s data would be unstructured, including emails, social media posts, and multimedia content. This prediction has now become a reality, emphasizing the growing need for businesses to effectively store, process, and analyze unstructured data.
Key Features of Unstructured Data
- Format: Freeform data that does not conform to a tabular structure (e.g., text documents, videos, audio files).
- Examples: Emails, social media posts, customer reviews, videos, images, sensor data.
- Storage: Data lakes, NoSQL databases, and cloud storage solutions.
Practical Use Cases
Unstructured data is essential for tasks that require analysis of large, diverse, and complex datasets. Use cases include:
- Sentiment Analysis: Analyzing social media or customer feedback to understand public opinion.
- Medical Imaging: Analyzing X-rays, MRI scans, and other images in healthcare.
- Video Surveillance: Processing video footage to identify security threats or patterns.
Key Differences Between Structured and Unstructured Data
Understanding structured data vs unstructured data is crucial for businesses managing large-scale information. The approach to data collection plays a vital role in determining how organizations store, process, and analyze data. Different types of data require tailored collection methods, which affect storage infrastructure, analysis tools, and overall scalability. Below, we compare structured and unstructured data, highlighting their fundamental differences.
In a Reddit thread discussing when to use structured vs. unstructured databases, users share differing views on data modeling. One key takeaway is that the database choice should depend more on the type of data rather than simply whether it's likely to change. Relational databases (RDBMS) like SQL can handle unstructured data, especially with formats like JSON, challenging the traditional notion that RDBMS are only suited for structured data. Additionally, some users caution that unstructured databases should be considered only when necessary, as they often require more complex infrastructure.

Bridging the Gap: Semi-Structured Data
While the differences between structured and unstructured data are clear, many businesses encounter semi-structured data, which falls in between. This type of data has some organizational properties but does not strictly follow a tabular format.
Here are some examples of semi-structured data:
- JSON or XML files that store data with predefined tags or attributes.
- Emails that contain structured metadata but unstructured message content.
- Log files that contain structured time stamps but freeform data in the body.
Challenges and Considerations in Data Collection
As businesses collect increasing amounts of structured and unstructured data, they face several challenges in storage, processing, security, and cost management. While structured data is easier to store and analyze, unstructured data requires more advanced tools and infrastructure to extract value. Understanding these challenges helps organizations develop effective data collection strategies that optimize both efficiency and compliance.
Storage & Management
Storing and managing unstructured data can be a significant challenge due to its large size and the variety of formats. Businesses must invest in solutions like data lakes or NoSQL databases to handle these large datasets. Structured data, on the other hand, can be efficiently stored in relational databases, which are easier to maintain and manage.
Processing & Analysis
Structured data is easier to query and analyze due to its organization, while unstructured data demands advanced technologies such as AI, machine learning, and NLP to uncover valuable insights. This complexity increases both the cost and effort involved in processing unstructured data.
Leveraging AI-powered analytics enhances the ability to derive meaningful insights from unstructured data, driving decision-making across industries
Cost & Infrastructure
Handling unstructured data often requires specialized infrastructure, which can be expensive. The cost of storage, processing, and analysis for unstructured data can exceed that of structured data due to its complexity and the need for advanced tools.
Security & Compliance
With the increasing volume of data being collected, both structured and unstructured data must adhere to data protection regulations such as GDPR and CCPA. Ensuring the privacy and security of both data types is essential, but unstructured data, due to its complex and varied nature, may pose greater challenges for encryption and access control.
Unlocking Data’s Full Potential with Sapien
As businesses collect and analyze more diverse data types, it becomes crucial to have a platform that can handle both structured and unstructured data efficiently. Sapien provides a seamless solution for businesses seeking to optimize their data collection processes, offering a decentralized network of human AI trainers to annotate and label both structured and unstructured data. With Sapien, companies can unlock valuable insights from their data, driving better decision-making and innovation.
Explore Sapien’s tailored data collection solutions today - whether you need to analyze structured data or harness the power of unstructured data. Schedule a consultation and learn how our AI-driven platform can optimize your data processes.
FAQs
How is unstructured data processed?
Unstructured data requires advanced AI and machine learning techniques, such as natural language processing (NLP) and computer vision, to extract useful insights.
Can structured and unstructured data be used together?
Yes! Semi-structured data, like JSON or XML, serves as a bridge between structured and unstructured data, allowing businesses to integrate both types for more comprehensive analysis.
Why is unstructured data important?
Unstructured data often contains valuable insights into customer sentiment, brand reputation, and market trends that structured data cannot capture.
How can Sapien help with data collection?
Sapien provides a decentralized workforce and AI-powered tools to annotate and process data, ensuring high-quality insights for businesses across various industries.