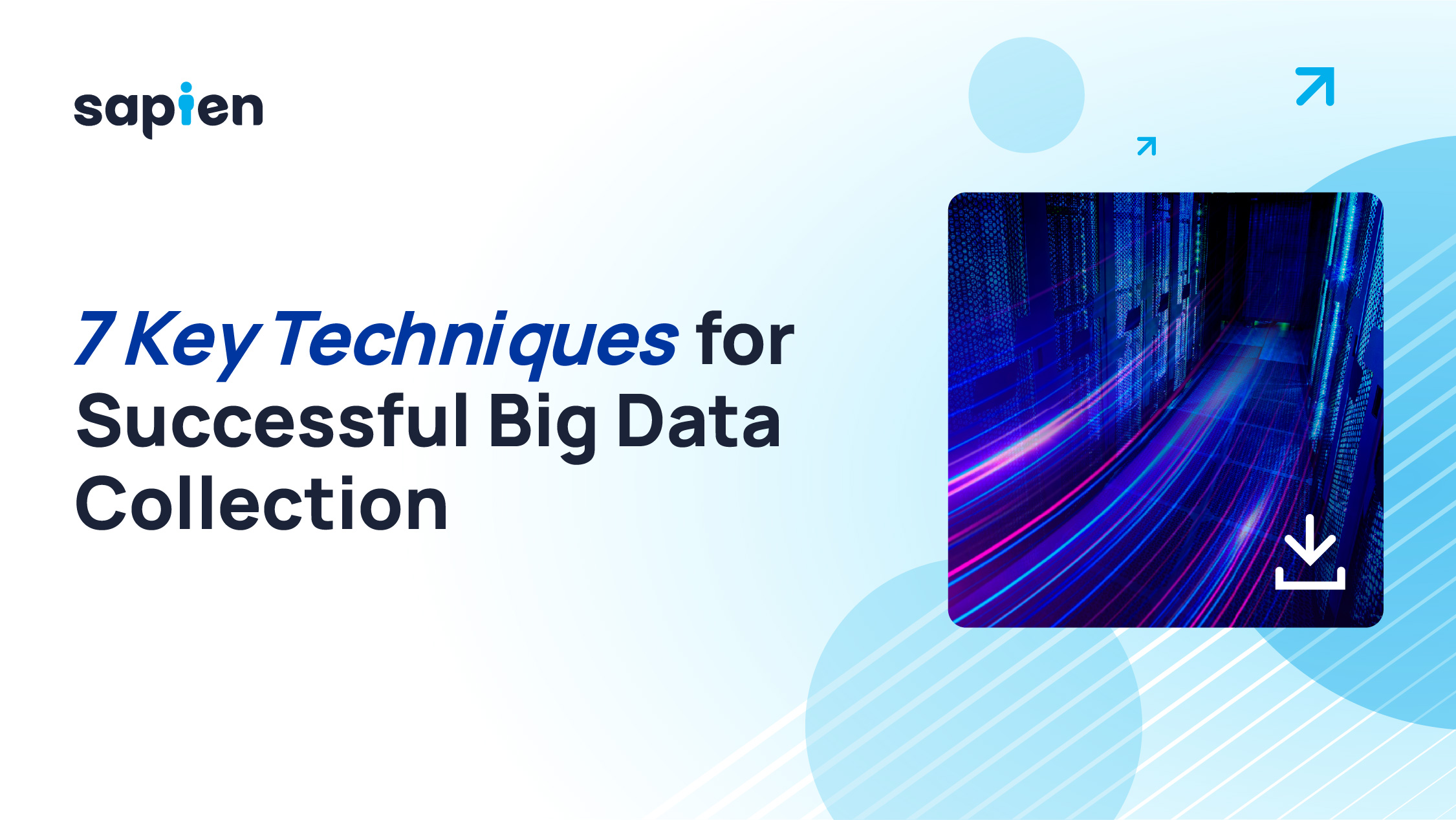
Big data collection is essential for driving business intelligence, product development, and growth. Whether it's predicting customer behavior or optimizing supply chains, data plays a central role in making decisions that shape entire industries. However, collecting data on such a large scale is no simple task - it requires precision, flexibility, and the right combination of tools and technologies to get it right.
This article breaks down seven key techniques for successful big data collection, offering actionable insights for organizations looking to transform raw data into real value.
Key Takeaways
- Automated Data Collection: Automating the data collection process helps businesses reduce human errors, increase operational efficiency, and handle large data volumes effortlessly.
- Scalability: Automated data collection ensures systems can scale with your business growth, managing increasing data influx without sacrificing performance.
- Real-Time Monitoring: Constant monitoring of automated data systems guarantees data quality, minimizes errors, and allows for quick adjustments to optimize workflows.
- Continuous Optimization: Regular assessments and improvements of the data collection systems help businesses maintain efficiency, adapt to changing needs, and stay ahead in their data-driven strategies.
Understanding Big Data Collection
Big data collection refers to the systematic process of gathering vast and diverse datasets - both structured (like databases) and unstructured (like social media posts or sensor data) - from multiple sources for analysis and insight generation.
How is big data collected? The answer lies in a variety of methods and tools, ranging from automated data pipelines to real-time streaming, IoT sensors, and API integrations. Collecting big data is not just about volume; it’s about velocity, variety, and veracity. Here are some common big data problems:
- Accuracy & Quality: Poor data leads to poor insights.
- Scalability: Systems must scale to handle growing data volumes.
- Security & Compliance: Sensitive data must be protected and regulated.
- Data Silos: Fragmented data sources can hinder unified analysis.
Understanding these challenges is essential before implementing a successful data collection strategy.
The 7 Key Techniques for Successful Big Data Collection
To build an effective data strategy, companies must go beyond ad-hoc data gathering methods and implement these key techniques that enable automation, speed, flexibility, and compliance.
1. Automated Data Pipelines
Manual data entry is time-consuming and error-prone. Automated big data collection methods solve this by programmatically gathering, processing, and routing data from one system to another. These pipelines manage ETL (Extract, Transform, Load) processes and ensure seamless data flow between sources.
If your organization deals with multi-source data or complex transformation rules, an automated pipeline is a must-have.
2. Real-Time Data Streaming
While batch processing is useful for periodic reporting and analysis, it falls short when immediate action is required. Real-time data streaming addresses this gap by enabling systems to process and analyze data the moment it is generated. This allows businesses to make time-sensitive decisions based on live insights.
For example, in finance, real-time analytics help detect fraudulent transactions the moment they occur. In healthcare, streaming data from medical devices enables real-time patient monitoring, potentially saving lives. In e-commerce, retailers can dynamically adjust pricing or show personalized offers based on a user’s live behavior.
Real-time big data collection techniques reduce decision latency and enable proactive interventions, rather than reactive analysis.
3. API Integrations and Web Scraping
APIs enable seamless data exchange between applications, providing real-time, structured data from platforms like Google Analytics, Salesforce, or Twitter. They offer scalable and consistent data access, making them ideal for aggregating information from multiple SaaS platforms.
When no official API exists, businesses may use web scraping to extract data from websites using scripts or automated tools. While powerful, it must be used ethically and legally, respecting site policies (e.g., robots.txt), avoiding excessive server load, and complying with data privacy laws.
Both methods expand data collection capabilities, allowing organizations to access structured and unstructured data efficiently.
By combining API integrations for structured data and web scraping for publicly available content, organizations can build comprehensive datasets that drive more accurate insights and stronger AI systems.
4. IoT and Sensor-Based Data Collection
The rise of Internet of Things (IoT) devices has revolutionized how data is collected from the physical world. Sensors embedded in machinery, vehicles, and consumer devices generate streams of data that can be used for predictive maintenance, behavioral analytics, and operational optimization.
In smart cities, for example, sensors monitor traffic patterns, air quality, and energy usage, helping planners improve urban infrastructure. In healthcare, wearable devices continuously track patient vitals, enabling early intervention. In manufacturing, sensors provide data on machine performance, helping prevent downtime.
Handling this high-frequency, time-series data requires specialized infrastructure. Edge computing - processing data closer to where it is generated - is often used to reduce latency and bandwidth usage before data is sent to the cloud for storage and analysis.
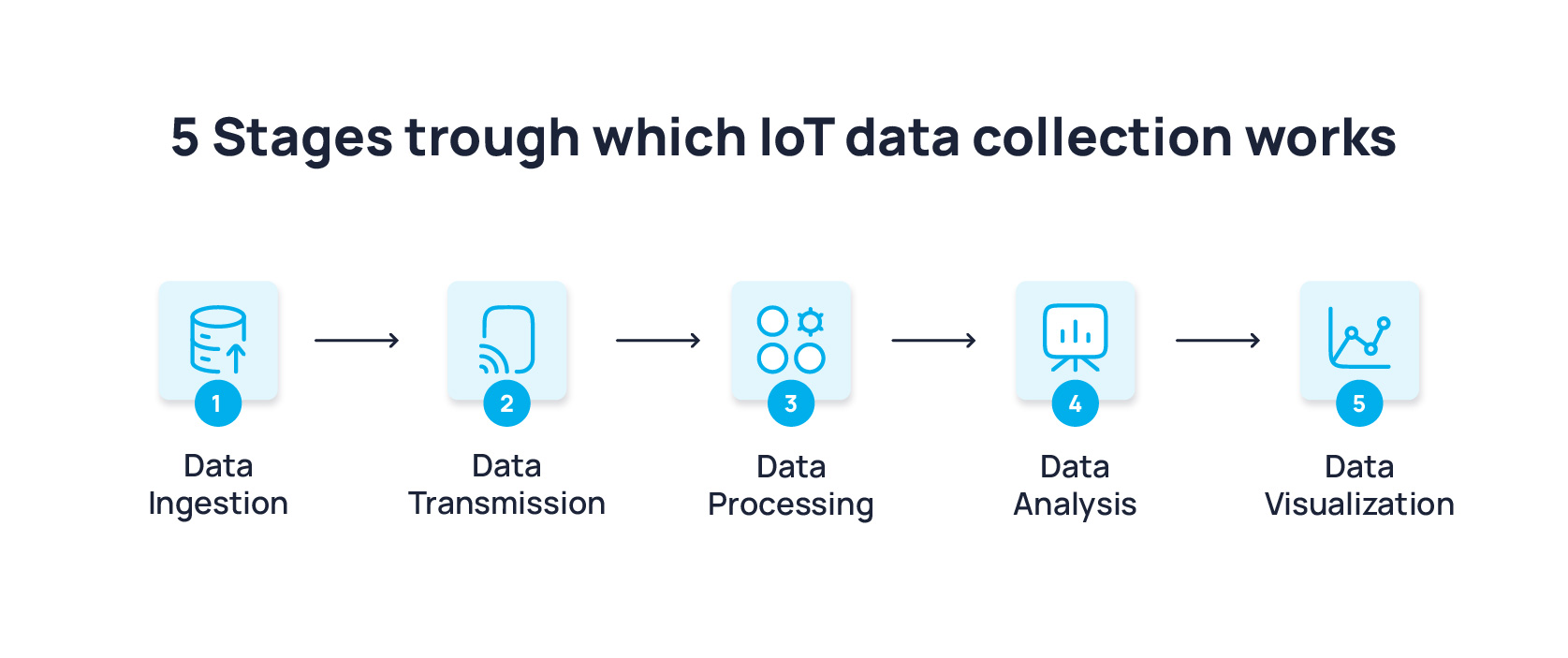
5. Data Governance and Compliance
As regulations around data privacy become more stringent, companies must adopt strong data governance frameworks. These frameworks ensure that data is collected, stored, and used in a way that meets legal, ethical, and operational standards.
Key compliance mandates include:
- GDPR (Europe): Requires user consent and data minimization.
- CCPA (California): Grants consumers control over personal information.
- HIPAA (US healthcare): Sets data security standards for medical records.
Effective data governance includes defining data ownership, maintaining audit trails, and enforcing access controls. This not only reduces legal risk but also builds trust with users and partners.
6. Cloud-Based Data Storage and Management
Cloud platforms offer unparalleled scalability, reliability, and cost-efficiency for storing and managing big data. With providers like AWS, Google Cloud, and Azure, organizations can dynamically scale storage and computing resources based on demand, eliminating the need for costly on-premise infrastructure.
Data storage models include:
- Data Lakes: Ideal for storing large volumes of raw, unstructured data.
- Data Warehouses: Optimized for analytics on structured data.
For example, AWS S3 is commonly used as a data lake, while Amazon Redshift and Google BigQuery serve as powerful, fast query engines for structured datasets.
Security remains a priority - cloud providers offer built-in encryption, multi-factor authentication, and detailed access logs. Proper role-based access control (RBAC) ensures only authorized users can access sensitive data.
7. AI-Driven Data Cleaning and Preprocessing
Once data is collected, it must be cleaned before it can be analyzed. This process includes removing duplicates, filling in missing values, standardizing formats, and flagging anomalies. Manual cleaning is not only time-consuming but also prone to human error, making AI-driven data preprocessing essential.
AI and machine learning streamline data cleaning by automating tedious tasks, improving both speed and accuracy. Research shows that errors in data can significantly degrade machine learning model performance. However, systematic data cleaning can enhance prediction accuracy by up to 52 percentage points, highlighting the importance of high-quality data. AI-powered tools efficiently detect inconsistencies, correct formatting issues, and validate data integrity, ensuring datasets are both reliable and actionable.
AI and machine learning not only automate data cleaning but also reduce the risk of errors that could impact model outcomes
Besides, Natural Language Processing (NLP) plays a crucial role in structuring unstructured text data. This enables applications such as sentiment analysis, customer feedback systems, and AI training to derive meaningful insights from text-based information. AI-based preprocessing ensures that data is not just abundant but also trustworthy - allowing organizations to make more accurate, data-driven decisions.
Best Practices for Effective Big Data Collection
While tools and techniques are important, strategic practices ensure long-term success:
- Enforce data standards across teams to avoid chaos and redundancy.
- Encrypt data at rest and in transit to protect against breaches.
- Track metadata to make datasets easier to find, trust, and reuse.
- Regularly audit systems for compliance and performance.
- Invest in team training to foster a data-centric culture.
A strong foundation in these practices amplifies the ROI of big data initiatives.
Optimizing Big Data Collection with Sapien
At Sapien, we specialize in custom data collection and labeling for organizations building high-performance AI models. Whether you're working with massive datasets for autonomous driving, patient diagnostics, or financial forecasting, we help you collect accurate, contextually rich data through expert-in-the-loop processes.
Sapien’s platform empowers enterprises with:
- Tailored workflows for domain-specific data collection
- Real-time feedback loops for continuous improvement
- Scalable operations without sacrificing accuracy or compliance
Our human-in-the-loop approach ensures that data quality keeps up with quantity - because in today’s AI-driven world, data is only valuable if it’s reliable. Get in touch with Sapien to elevate your data collection strategy.
FAQs
What is the difference between structured and unstructured data?
Structured data fits neatly into rows and columns (e.g., Excel spreadsheets), while unstructured data includes emails, PDFs, social media posts, images, and videos - data that doesn’t follow a predefined format.
How do you choose between a data lake and a data warehouse?
Use a data lake when you want to store raw, diverse datasets for flexible access. Opt for a data warehouse when your use case requires fast, reliable queries of structured data.
How can small businesses benefit from big data collection?
By leveraging cloud tools, automation, and freemium APIs, even small businesses can gather customer behavior data, optimize marketing campaigns, and personalize services without breaking the bank.