The Key to AI Success? High-Quality Training Data for AI
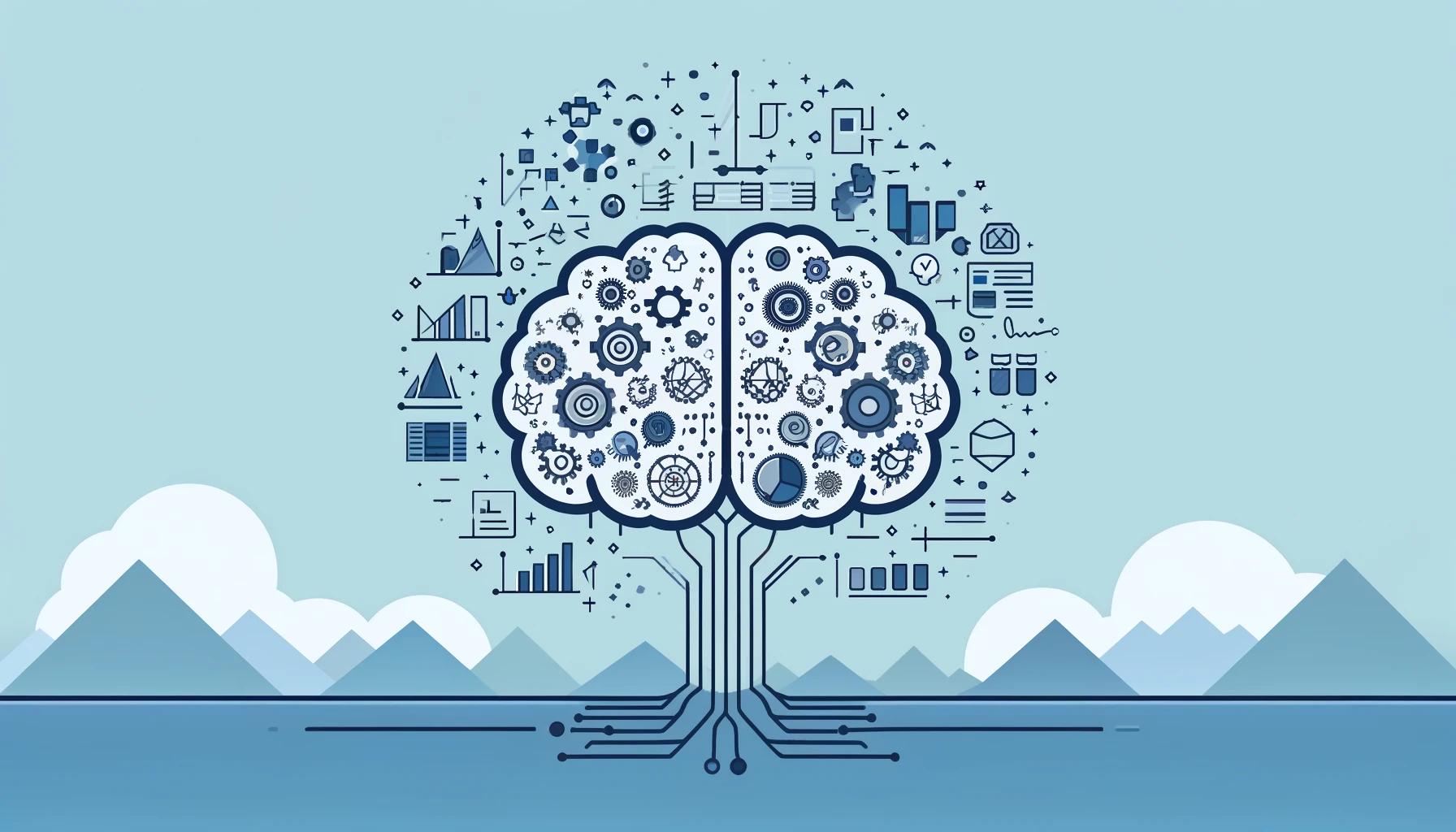
When it comes to artificial intelligence (AI), the data used to train machine learning models is arguably more important than the algorithms themselves. Without quality training data, even the most advanced AI techniques will fail to produce accurate insights, or build trustworthy AI. As advances in AI unlock new possibilities across industries, the hunger for more (and better) training data continues to intensify.
What exactly constitutes training data? And why is sourcing and labeling high-quality datasets so critical for developing fair, responsible, and useful AI systems? Let’s explore the role of training data in AI and why it deserves more attention as a top priority for anyone leveraging AI.
What is Training Data?
A Foundation for Machine Learning Models
Training data refers to the data used to teach a machine learning model to correctly interpret and handle new data. It is the basis for creating AI systems that can make predictions, translate languages, identify patterns, and much more. Training data is used by data scientists to both develop and test machine learning algorithms.
Training data consists of examples that are labeled or annotated to indicate the ideal output or prediction from the AI model when analysing that input data. These labels help the algorithm learn over time via pattern recognition until it can start making accurate predictions when presented with never-before-seen data.
For example, imagine building an image recognition model to automatically identify different types of animals. The training data would consist of a diverse range of images depicting various animals correctly tagged with labels indicating “cat”, “dog”, “bird”, etc. By learning the patterns from this labeled data, the model can recognize these animals when presented with new images.
The better and more representative the training data, the better the developed AI system performs in the real world. That’s why curating high-quality training data for AI through careful labeling and sampling techniques is so important for AI success.
Data Labeling for AI Models
The Importance of Human Feedback in Training AI
At the core of creating quality training data is the need for humans to manually label and annotate raw data to indicate the desired outputs or predictions. Although machine learning promises more automated insights from data, humans currently play an irreplaceable role in overseeing and guiding AI to ensure it aligns with real-world needs and constraints.
This is especially true in complex AI models, such as diffusion models, which require precisely labeled and high-quality datasets to perform tasks like image generation or natural language processing. Labelling data is tedious, time-consuming, and expensive work involving large teams of human annotators from services like Appen and Scale AI. However, their contextual understanding and judgment are indispensable for training performant and responsible AI systems.
Techniques to Scale Up Labeling
Several techniques help ease the bottlenecks in AI training data labeling:
- Platforms like Sapien break labeling work into micro tasks performed by thousands of workers
- Assisted labeling combines manual work with machine learning to semi-automate parts of the process
- Inferential labeling uses models to propagate labels from small labeled datasets to unlabeled data
While promising, these still require human oversight and quality checks to catch anomalies and ensure high accuracy. Standards like ISO 20252 guide the best practices in data labeling to uphold quality. As models depend wholly on training data, accuracy problems compound rapidly if labels are misleading or biased.
The Growing Need for Training Data in AI
Ever-Growing Data Hunger of AI Systems
As AI capabilities grow more advanced in areas like computer vision, natural language processing and robotic control, their appetite for data expands correspondingly. State-of-the-art models can require hundreds of times more parameters and data than predecessors from even a few years ago.
For example, the GPT-4 model trained by AI research company OpenAI on enormous volumes of text data demonstrates human-like language proficiency unmatched by previous attempts. However, it also raises questions about the feasibility of scaling current data labeling pipelines to sustain such data-intensive models.
Creating Diverse, Unbiased Datasets
Not just quantity, but diversity and balance are vital attributes for curating responsible training data. Models trained on narrow demographic data risk perpetuating and amplifying those biases on overlooked groups. Mitigating unfairness requires datasets spanning diverse geographical regions, demographics, ethnicities, genres and more.
Careful dataset design strives to represent all groups appropriately at both training and testing stages. We are still early in our understanding of how to create genuinely fair and helpful AI. But emphasizing responsible data practices is a step in the right direction.
Responsible, Lawful, Helpful, Fair AI Through RLHF
Aligning AI Systems to Ethical Values
As AI is increasingly deployed to automate decisions in areas like healthcare, finance and criminal justice, we need assurance these systems align with moral and legal principles before real-world rollout. Fields like machine learning often focus overwhelmingly on performance metrics like accuracy. However, optimizing only for narrow technical definitions of performance risks unintended consequences.
Issues from Poor Quality or Biased Data
Several high-profile cases exist already where questionable real-world system behavior traced back to deficiencies in training data:
- Racial bias in risk assessment tools predicting likelihood of recidivism
- Facial analysis tools with much higher error rates for women and darker skin tones
- Toxic, extremist language models like Tay from Microsoft
In each case, the models reflected - and amplified - the biases and imperfections of the data used to develop them. Ethical AI goes beyond merely avoiding direct discrimination. It requires a holistic assessment of how systems might indirectly contribute toward adverse outcomes for disadvantaged groups when deployed carelessly at scale.
Techniques to Build More Ethical AI
Thankfully techniques do exist to audit datasets and models more rigorously:
- Quantifying demographic variance between training and real-world deployment data
- Testing model performance across subgroups to catch uneven effects
- Adversarial attacks to reveal blindspots and hidden failure modes
- Simulating model decisions over simulated population samples
Creating High-Quality Training Data Sets
Compiling Diverse and Representative Data
High-quality training data must sufficiently represent all expected real-world conditions seen at test time. However, most published training datasets cover only narrow slices of reality. Consider an autonomous vehicle model trained only on daytime driving data. Failure to experience night, rain and other conditions during training mean the model cannot handle those scenarios reliably.
For assembling rich training data, techniques like web scraping, crowdsourcing and aggregating multiple datasets help capture diversity often lacking in single-source data. However, this introduces the challenge of merging datasets with very different characteristics. Ensuring coherence requires steps for resolving conflicts, normalizing labels, handling missing data and aligning distributions statistically.
Maintaining Datasets over Time
Unlike static assets, dataset value depends on keeping pace with a changing outside world. Regular updating ensures datasets continue reflecting true population statistics tracked by census surveys. Versioning also aids reproducibility in AI research by preserving old dataset snapshots used in publications.
The Future of Training Data for AI Lies in Data Labeling Services
Automating Parts of the Pipeline
While integral today, manually labeling vast volumes of AI training data cannot economically scale long-term. The field urgently needs to reduce reliance on human annotation through ML techniques like semi-supervised learning, generative adversarial networks, reinforcement learning and neuro-symbolic approaches combining neural nets with reasoning algorithms.
Synthetic Data Generation
Synthetically generating realistic artificial training data holds promise for expanding dataset diversity cheaply without paying the labeling cost. Smart augmentation techniques transform real-world seed data into plausible new variants usable for training purposes even if it does not match naturally occurring data exactly.
An Ongoing Need for Human Oversight
Yet while these innovations might lessen data labeling demands in future, none eliminate the need for human oversight over training data practices yet. Humans have the sole capacity for critiquing potential social impacts from deploying AI systems built atop training data. Keeping humans in the loop remains essential even as parts of the pipeline shift toward automation.
The Competitive Edge from Quality Training Data for AI
As AI capabilities continue advancing rapidly across industries, access to quality training data is increasingly the key competitive edge. Companies sitting on useful data or with resources to procure and label such data will stand better positioned to capitalize as leaders in the next wave of AI growth.
However, emphasizing the quantity of data alone risks unintended harm if aspects like diversity, balance and ethical alignment get neglected inadvertently. Responsible and effective deployment of AI requires holistic oversight spanning the full pipeline - from raw data curation to model development, evaluation, monitoring and maintenance.
Get in Touch with Sapien to Learn More About Data Labeling Services for LLMs and Label Your Training Data for AI Models
To learn more about how Sapien can fulfill your unique enterprise-level data labeling and fine-tune LLM models at scale, contact our team today to book a demo. Our global network of domain experts can annotate complex text, image, video and audio data to train performant AI systems including:
- Language models for some text
- text classification
- Summarization
- sentiment analysis
- Dialogue
- and more
- Computer visionsome text
- Segmentation
- object detection
- image recognition
- and more
With Sapien, you get reliable access to multi-domain annotation skills backed by an enterprise-grade quality assurance process. This frees your team to focus their specialized expertise on high-value tasks like model development and deployment.
We employ encryption, access controls and auditing to keep sensitive data secure as it flows through our human-in-the-loop data annotation pipeline spanning the globe. Contact us to get a custom quote and book a demo to experience the Sapien platform today!