The Growing Demand for Data Labeling Professionals and the Challenges of In-House Labeling
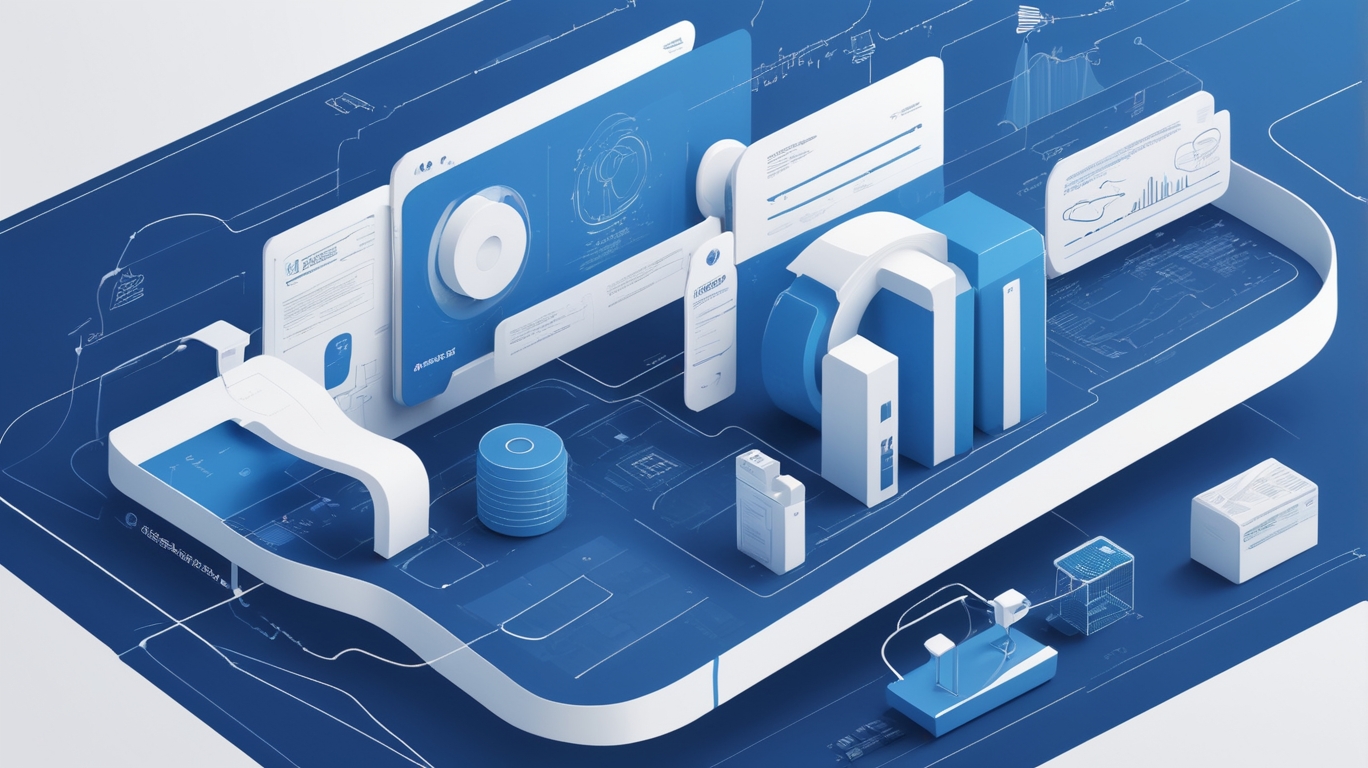
In recent years, the exponential growth of data, coupled with the widespread adoption of artificial intelligence (AI) across industries, has fueled an unprecedented demand for skilled data labeling professionals. As companies increasingly rely on AI to drive innovation and efficiency, the need for high-quality labeled data to train machine learning models effectively has become more critical than ever. However, many company are struggling with the challenges of in-house data labeling, which can be inefficient, costly, and less effective compared to outsourcing to specialized data labeling companies.
The global data annotation and labeling market size was valued at USD 0.8 billion in 2022 and is expected to grow at a staggering compound annual growth rate (CAGR) of 33.2% from 2022 to 2027. This rapid expansion is driven by the increasing complexity of datasets, the emergence of advanced data labeling tools, and the rising prominence of AI and machine learning across sectors. North America currently dominates the data labeling market due to high technological adoption, the presence of major players, and significant investments in AI and machine learning. However, the Asia Pacific region is also experiencing rapid growth, thanks to increasing technological investments in countries like China, Japan, and India, as well as the region's large workforce, which makes it a hub for manual data labeling services.
Despite the growing demand for data labeling professionals, many organizations are struggling with the inefficiencies and challenges of in-house data labeling. One of the primary issues is the cost and resource intensity associated with maintaining an in-house data labeling team. Significant upfront investments in infrastructure, training, and personnel are required, which can be a substantial financial burden for many organizations. Additionally, in-house teams may not have the specialized expertise needed for specific data labeling tasks, such as computer vision or natural language processing, leading to lower quality labels and reduced model performance.
Scalability and flexibility are also major concerns for in-house data labeling teams. As data labeling needs change, particularly during peak periods, in-house teams can struggle to scale resources effectively, resulting in delays and inefficiencies. Outsourcing data labeling to specialized service providers allows organizations to focus on their core competencies, rather than diverting resources to labeling tasks that are not their primary expertise.
Data security is another critical issue that organizations must consider when deciding between in-house and outsourced data labeling. In-house data labeling can raise data security concerns, as sensitive data is handled internally. By outsourcing to a reputable provider, organizations can alleviate these concerns through the implementation of robust security measures and strict data handling protocols.
Quality control and feedback mechanisms are also essential for ensuring high-quality data labeling and annotation. In-house teams may not have the same level of quality control and feedback processes as specialized service providers, which can lead to lower quality labels and reduced model performance. Outsourcing to experienced data labeling companies ensures that rigorous quality control measures are in place, resulting in more accurate and consistent labels.
Managing large volumes of data is another challenge that in-house teams often face. As datasets continue to grow in size and complexity, in-house teams can struggle to efficiently manage and label vast amounts of data, leading to delays and inefficiencies. Specialized data labeling companies with crowdsourcing data annotation services, on the other hand, are equipped with the tools, expertise, and resources necessary to handle large-scale data labeling projects effectively.
Consistency and accuracy are critical factors in data labeling, as they directly impact the performance of machine learning models and the quality of decision-making. In-house teams may struggle to maintain consistency and accuracy across large datasets, particularly when dealing with complex or specialized labeling tasks. Outsourcing to experienced data labeling providers ensures that labels are consistent and accurate, thanks to their expertise, advanced tools, and rigorous quality control processes.
Finally, access to advanced tools and technologies is essential for delivering high-quality data labels efficiently. In-house teams may not have the resources or expertise to invest in and utilize cutting-edge data labeling tools and technologies, limiting their ability to deliver high-quality labels at scale. Specialized data labeling companies, on the other hand, continually invest in the latest tools and technologies to ensure that they can deliver the best possible results for their clients.
In conclusion, the demand for skilled data labeling professionals is rapidly growing as companies increasingly rely on AI and machine learning to drive innovation and efficiency. However, organizations must carefully consider the challenges and inefficiencies associated with in-house data labeling, including cost and resource intensity, limited expertise, scalability and flexibility issues, data security concerns, quality control and feedback limitations, time and effort requirements, difficulty in managing large volumes of data, challenges in ensuring consistency and accuracy, and limited access to advanced tools and technologies.
By outsourcing data labeling to specialized service providers, organizations can overcome these challenges and achieve more efficient and effective labeling processes. Specialized data labeling companies offer the expertise, resources, and advanced tools necessary to deliver high-quality labels at scale, allowing organizations to focus on their core competencies and drive innovation through AI and machine learning.
Unlock the Power of Your AI with Sapien's Expert Data Labeling Services
As the demand for skilled data labeling professionals continues to surge, companies are increasingly turning to specialized data labeling providers like Sapien to overcome the challenges and inefficiencies associated with in-house labeling. Sapien's data labeling services combine the power of human intelligence with cutting-edge AI technologies to deliver high-quality training data for your machine learning models.
With a global network of over 80,000 skilled labelers spanning 165+ countries and speaking 30+ languages and dialects, Sapien has the expertise and resources to handle data labeling projects of any size and complexity. Whether you need question-answering annotations, data collection, model fine-tuning, or test and evaluation services, Sapien's team of experienced subject matter experts is ready to become an extension of your team and deliver successful projects.
Sapien's flexible and customizable labeling solutions can be tailored to your specific data types, formats, and annotation requirements, ensuring that your machine learning models receive the high-quality training data they need to achieve optimal performance. By leveraging Sapien's expertise in text classification, sentiment analysis, semantic segmentation, and image classification, you can unlock the full potential of your AI and drive innovation across your organization.
Don't let data labeling bottlenecks hold you back. Schedule a consult with Sapien today and experience the difference that expert human feedback can make in your AI initiatives. Schedule a consultation with Sapien's team to learn more about how their scalable data labeling services can help you achieve your AI goals and stay ahead of the competition.