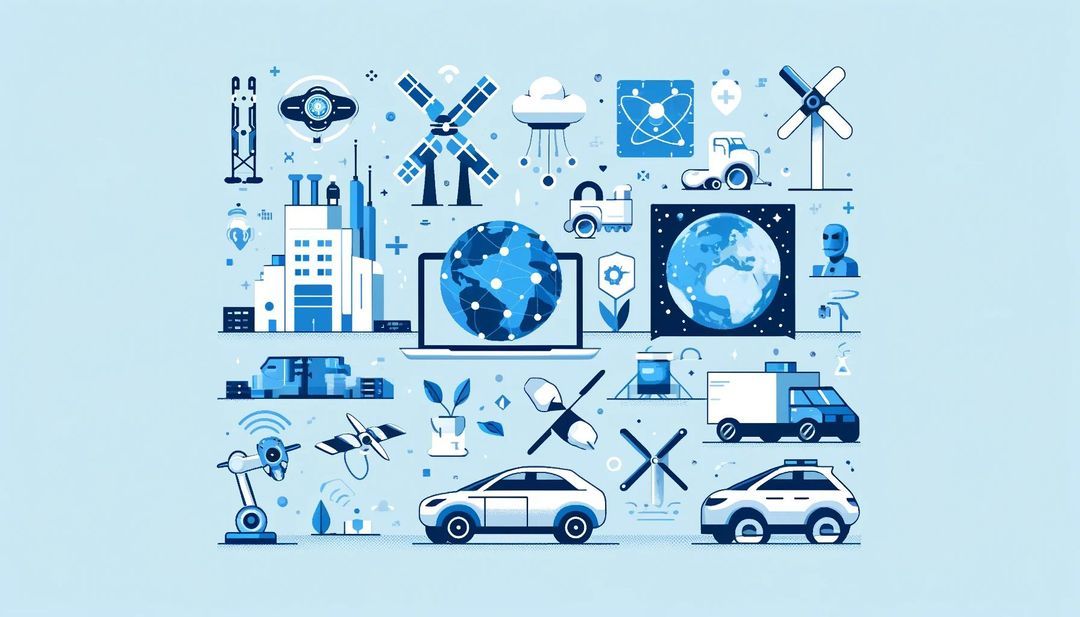
The artificial intelligence (AI) industry is experiencing a period of unprecedented growth, and we've seen the rapid development of increasingly sophisticated models. There has also been a shift in AI dominance from academia to industry, and a growing emphasis on responsible AI development. Let's discuss some of the trends shaping the AI landscape, the advancements in model development, and the escalating costs associated with AI research.
Advancements in Model Development and Evaluation
OpenAI's GPT-4 continues to dominate the AI rankings, showcasing exceptional performance across various tasks and metrics. However, newer entrants like Google's Gemini and Mistral AI's Mistral-Medium are gaining traction, demonstrating the competitive nature of the AI landscape. These models are evaluated based on their ability to complete tasks and are ranked on leaderboards that track their performance across various metrics. The AI Index Report highlights that AI has surpassed human performance on certain benchmarks, such as image classification and natural language processing, but still lags behind in more complex tasks like competition-level mathematics and visual commonsense reasoning.
The development of AI models has seen a significant shift, with industry surpassing academia in terms of output. In 2023, industry produced 51 notable machine learning models, while academia contributed only 15. This shift highlights the industry's growing dominance in AI research and development, fueled by greater resources, data, computing power, and funding.
Industry vs. Academia: A Changing Landscape of AI Research
Industry's dominance in AI research is further emphasized by the number of foundation models released, with cloud giants like Google and Microsoft leading in the creation and release of these models. Google has been at the forefront, releasing the most foundation models since 2019, followed by OpenAI, indicating the industry's leadership in AI model development.
While industry focuses on developing large and expensive AI models, academia plays a crucial role in ensuring that research results are publicly available, considering all types of research, and fostering vibrant research communities. Academic institutions also focus on ensuring that a diverse range of research is conducted, contributing to a broader spectrum of AI research.
Escalating Costs and the Rise of Open-Source Models
The training costs of state-of-the-art AI models have reached unprecedented levels, with OpenAI's GPT-4 using an estimated $78 million worth of compute and Google's Gemini Ultra costing $191 million. The exponential growth in the size and cost of AI models is evident, reflecting the increasing complexity and sophistication of these systems.
Despite the industry's resource advantage, there is a trend towards open-source foundation models. In 2023, 65.7% of newly released models were open source, compared to 44.4% in 2022. This trend democratizes access to AI technology, enabling researchers and developers with limited resources to leverage and contribute to AI advancements.
Responsible AI Development and Deployment
The AI community is increasingly focused on responsible AI development and deployment, with efforts to assess the impact of AI systems and mitigate risks for those affected. This includes addressing concerns related to bias, fairness, transparency, and accountability in AI algorithms and decision-making processes.
Factors Affecting the Costs of Training AI Models
The costs associated with training AI models are influenced by several factors:
- Hardware: AI models require specialized high-end hardware like GPUs and TPUs for high-performance computing workloads. These hardware components are essential for processing large volumes of data and computations, but their high costs contribute significantly to the overall expense of training AI models.
- Software: The costs associated with AI software training can be reduced through increased efficiency and scalability. Software frameworks like TensorFlow and PyTorch enable developers to train complex deep learning models on distributed systems with high performance, ultimately saving time and resources.
- Cloud-Based Artificial Intelligence: Cloud-based AI training offers scalable computing resources on demand, reducing costs by providing a pay-as-you-go model. Cloud providers also offer pre-built AI services that accelerate AI training processes, making it more cost-effective for businesses to access the computing power needed for training AI models.
Partner with Sapien to Improve Your AI Models
As the technical complexities of AI model development continue to evolve, ensuring high-quality training data and efficient labeling processes is more crucial than ever. Sapien, with its expertise in data collection, labeling, and fine-tuning through human feedback, can help you navigate these complexities and unlock the full potential of your AI models.
Whether you're developing cutting-edge transformer models for natural language processing or training convolutional neural networks for computer vision tasks, Sapien can provide the human-in-the-loop labeling process that delivers real-time feedback for fine-tuning your datasets. This ensures the highest level of accuracy and performance for your AI models.
Why Choose Sapien?
- Accuracy and Scalability: Sapien's data collection and labeling services focus on accuracy and scalability, ensuring that your AI models are trained on high-quality data that meets your specific requirements.
- Efficient Labeler Management: Sapien's labeler management allows for segmented teams, so you only pay for the level of experience and skill sets your data labeling project requires.
- Fine-Tuning through RLHF: Sapien provides precise data labeling with faster human input to enhance the robustness and input diversity of your LLMs, improving their adaptability for your enterprise applications.
- Scale Labeling Resources Quickly: Sapien can quickly scale labeling operations up and down for annotation projects of all sizes, providing human intelligence at scale.
- Labeling Flexibility and Customization: Sapien can customize labeling models to handle your specific data types, formats, and annotation requirements.
A Flexible Team to Support Your Labeling Journey
Sapien has the expertise and operational scalability to find the labeling expertise you need for any project. Whether you require labelers fluent in specific languages or experts in niche fields, Sapien's internal team can help you scale quickly.
Enrich Your LLM's Understanding of Language and Context
Sapien combines AI and human intelligence to annotate all input types for any model. Whether it's question-answering annotations, data collection, model fine-tuning, testing & evaluation, text classification, sentiment analysis, semantic segmentation, or image classification, Sapien can help you enhance your LLM's understanding of language and context.
Take the Next Step with Sapien
Don't let data labeling bottlenecks hinder your AI development. Partner with Sapien to leverage the power of human feedback and build the most performant and differentiated AI models. Schedule a consult today to learn how Sapien can build a scalable data pipeline for you.