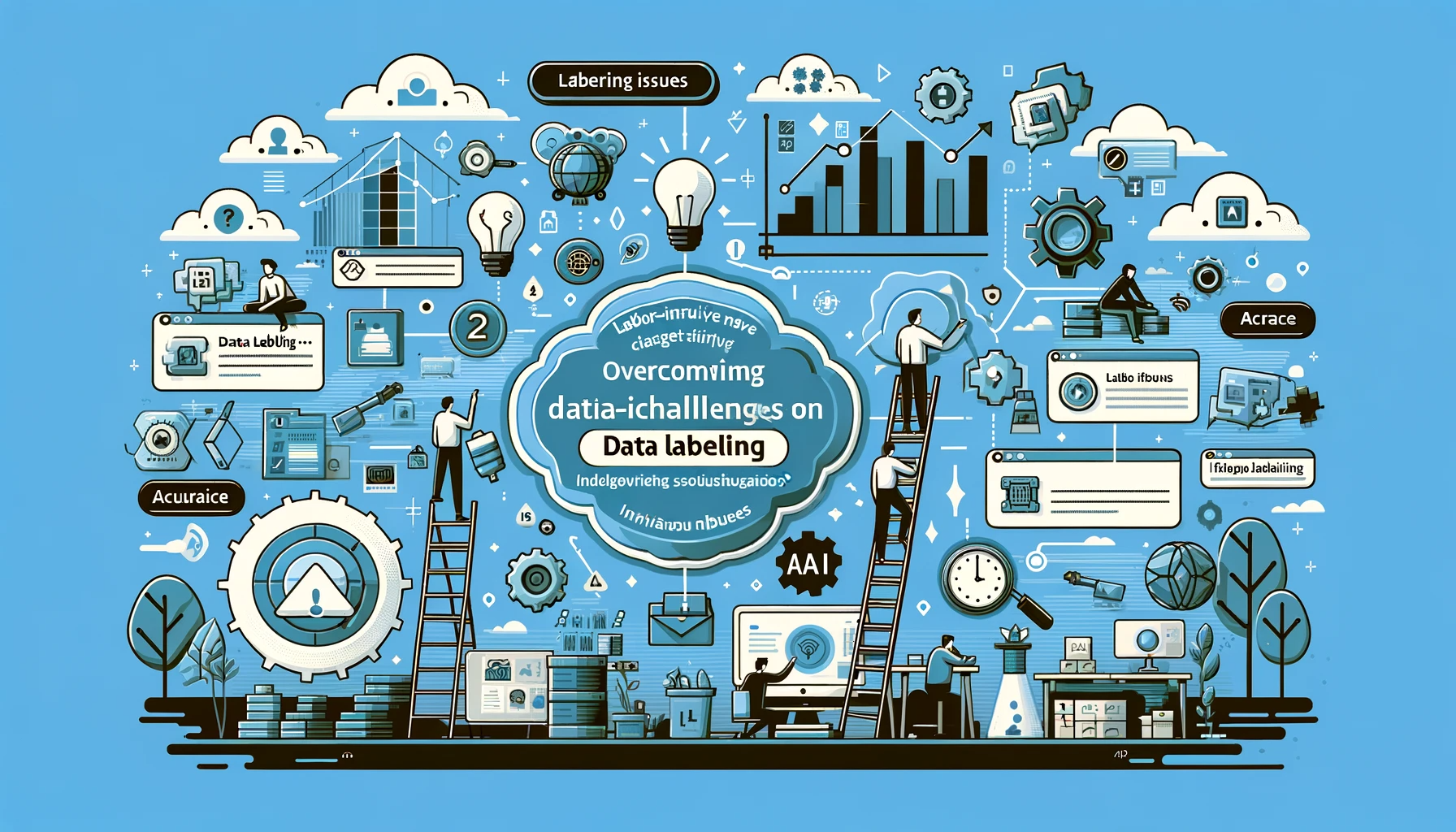
Data labeling, at its most basic, involves the annotation of data to make it comprehensible for AI models. This step is crucial for training machine learning algorithms, but it's still filled with challenges that can significantly affect the effectiveness and accuracy of AI systems. Understanding these challenges is the first step in addressing them effectively with scalable data labeling services.
The Labor-Intensive Nature of Data Labeling
One of the most significant challenges in data labeling is its labor-intensive nature. Traditionally, data labeling has required extensive human input. Labelers must meticulously go through vast amounts of data, making it a time-consuming and expensive process. This challenge is magnified when dealing with complex data types or when high levels of precision are required. The manual effort involved not only escalates costs but also introduces the risk of human error, potentially leading to inconsistencies in the labeled data.
Addressing Accuracy Issues in Data Labeling
Accuracy in data labeling is paramount. Inaccurate labeling can lead to poor training of AI models, resulting in biased or ineffective AI systems. Ensuring the accuracy of labels is challenging, especially when dealing with subjective or nuanced data. Moreover, maintaining consistency in labeling across different labelers and over large datasets is a complex task that can directly impact the quality of AI training.
Innovative Approaches to Data Labeling
The AI industry is actively seeking solutions to these challenges. One approach is the development and adoption of sophisticated tools that utilize AI to assist in the data labeling process. These tools can automate parts of the labeling process, significantly reducing the time and labor required. However, automation brings its own challenges, including the need for initial training data and the risk of perpetuating existing biases.
Another approach is crowdsourcing, which involves distributing the labeling task across a large number of people, often through online platforms. Crowdsourcing can enhance scalability and speed up the labeling process. It also introduces diversity in the labeling process, which can help in reducing bias. However, managing quality and consistency in crowdsourced labeling requires robust quality control mechanisms.
In addition to these, active learning is another technique gaining traction. This approach involves training the AI model on a small amount of labeled data and then using the model itself to identify the most informative data points for further labeling. This can make the labeling process more efficient by focusing human efforts on the most impactful data.
Emphasizing the Ongoing Evolution of Data Labeling Practices
The field of data labeling is continuously evolving, driven by the growing demands of the AI industry. The innovations in labeling techniques and tools are not only addressing the existing challenges but are also shaping the future of how AI systems are trained. The focus is increasingly on finding the right balance between human input and automation, ensuring both efficiency and quality in labeled datasets.
The challenges of data labeling in AI development are significant, but they are not insurmountable. By adopting innovative approaches and continually refining techniques, the industry can overcome these hurdles. The future of AI depends heavily on the effectiveness of data labeling, making it an area of critical importance and ongoing research.
Overcome Data Labeling Challenges with Sapien: Book a Demo
Tackling the labor-intensive and complex nature of data labeling in AI is crucial for success. Sapien addresses these challenges head-on, offering a unique platform that connects you with a diverse, global pool of labelers. Our two-sided marketplace for scalable data labeling can help your mid-market AI models bridge the gap with Big Tech, ensuring accuracy and diversity in data labeling. Take the first step towards overcoming data labeling challenges and enhancing your AI development with Sapien and book a demo.