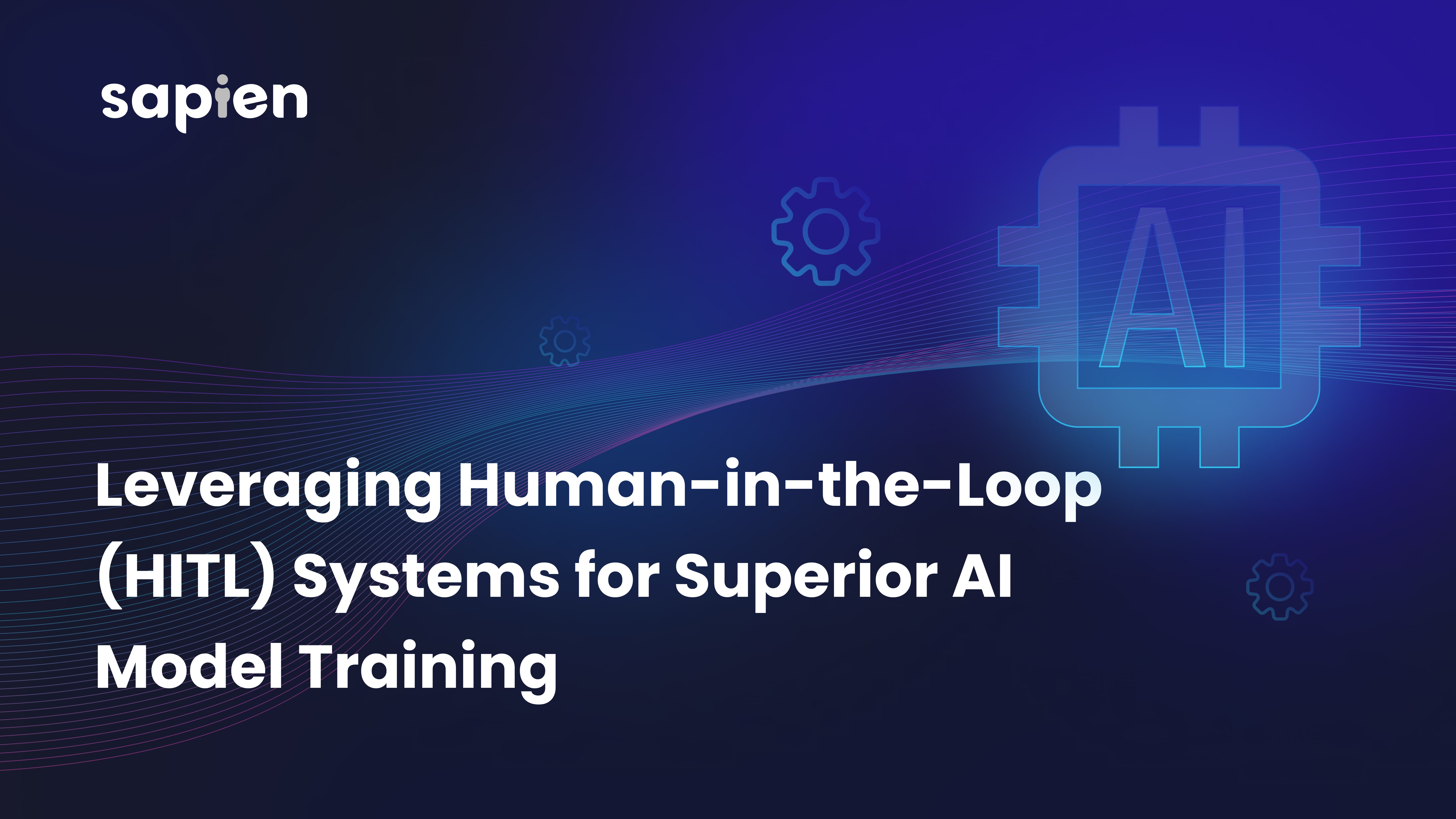
Human-in-the-Loop (HITL) systems are what help advance artificial intelligence (AI) and machine learning (ML) model training. Incorporating human oversight and input allows these systems to address critical issues of accuracy, reliability, and bias, which are particularly important in sensitive and highly regulated sectors such as healthcare, finance, and critical infrastructure. Here’s how data labeling techniques leverage human-in-the-loop systems for quality control measures to deliver meaningful, measurable improvements in data output.
What is Human-in-the-Loop (HITL)?
Human-in-the-Loop (HITL) systems are AI model training frameworks that involve human interaction during the development and deployment phases. HITL systems make sure that automated processes are continually reviewed and refined by human experts. This interaction enables the identification and correction of errors, biases, and other nuances that purely automated systems might otherwise overlook.
In HITL, humans provide feedback and oversight at various stages of the AI model lifecycle. This can include initial data annotation, iterative feedback during model training, and real-time decision-making corrections during deployment. The goal is a more accurate, transparent, and fair AI model that aligns with human values and ethical standards, and delivers at or above the expected performance level.
Benefits of HITL Systems in AI Training
Human-in-the-loop (HITL) systems improve AI accuracy by incorporating expert feedback into model training. This leads to more reliable and adaptable AI solutions across various applications.
Improved Accuracy
Verifying and correcting automated annotations means that users can trust that the data used for training is accurate and reliable. This feedback loop helps in continuously improving the model’s performance and reducing the margin of error over time.
Bias Reduction
AI models can be prone to biases present in their training data. Human evaluators can identify these biases and mitigate their impact, leading to more equitable and fair AI decisions. This is particularly important in applications where decisions can significantly impact individuals, such as loan approvals or medical diagnoses.
Contextual Understanding
Humans have the ability to understand and interpret nuanced data that machines may misinterpret. This contextual understanding is important for handling complex or ambiguous situations, making sure that the AI system can make informed decisions even in scenarios not covered by the training data.
Implementing HITL Systems
Implementing Human-in-the-loop (HITL) systems involves integrating human expertise into the AI training process, ensuring that models learn from real-world feedback. This approach helps to refine algorithms, reduce errors, and create AI systems that better understand and adapt to complex tasks. Here's how to do it effectively.
Workflow Design
Integrating HITL into the AI training process requires a well-defined workflow:
- Data Collection and Annotation: Humans annotate the data, providing high-quality labeled datasets for initial model training.
- Model Training: The AI model is trained using the annotated data.
- Human Review and Feedback: Human experts review the model outputs, identify errors or biases, and provide feedback.
- Model Refinement: The model is retrained based on the feedback, improving its accuracy and reliability.
Feedback Loops
Continuous feedback loops are essential for HITL systems. As the AI model encounters new data and scenarios, human experts review the outputs and provide corrective feedback to keep the model accurate and adapt to new challenges over time.
Tooling and Platforms
Several tools and platforms facilitate HITL systems, including annotation tools for data labeling and feedback management systems to track and integrate human feedback. Companies like Sapien offer customized labeling modules and platforms for each project to ensure optimal data quality and seamless integration.
Challenges in HITL Systems
Challenges in Human-in-the-loop (HITL) systems include the need for continuous human involvement, which can be time-consuming and resource-intensive. Additionally, balancing human input with automation requires careful design to prevent biases and maintain the efficiency of the AI system, and is the key to AI success.
Scalability
Scaling human involvement for large datasets presents significant challenges. Effective HITL systems require efficient processes and tools to manage the volume of data and ensure consistent quality, including a decentralized workforce and scalable annotation platforms.
Consistency
Consistency is key. This can be addressed by implementing standardized protocols, rigorous training for human annotators, and continuous quality control measures.
Cost
Incorporating human feedback does involve additional costs for hiring and managing human resources. However, these costs are often justified by the significant improvements in model accuracy and reliability, which can lead to long-term efficiencies and cost savings.
Applications of Human-in-the-Loop Systems
Human-in-the-loop (HITL) systems find applications across various fields, enhancing AI performance in tasks that require nuanced decision-making. From improving medical diagnostics to refining customer service chatbots, HITL systems ensure that AI models benefit from human expertise, leading to more accurate and reliable outcomes.
AI Content Moderation
In AI content moderation, HITL systems ensure accurate data labeling by combining automated processes with human review. This approach guarantees that user-generated content is properly analyzed, and inappropriate material is efficiently removed.
Autonomous Driving
Human oversight ensures that labeled data used for training AI models accurately reflects real-world conditions, providing an additional layer of reliability and safety.
Healthcare
In healthcare, HITL systems in data labeling ensure that AI models are trained on accurately labeled medical data. Human reviewers help detect and correct errors, ensuring the AI can make precise and reliable diagnoses to maintain high standards of patient care.
Finance
Human oversight helps identify and correct biases and errors in financial AI models so AI-driven financial decisions are fair and compliant with regulations.
A More Powerful and Accurate Approach to Data Labeling
Human-in-the-Loop (HITL) systems are the most powerful approach to improving AI model training by integrating human oversight and feedback during the data labeling process. While there are challenges in scalability, consistency, and cost, the advantages of HITL systems in critical and regulated industries are substantial, and Sapien addresses those issues with a decentralized labeling workforce and management.
Improve Your AI Model Accuracy with Sapien's Human-in-the-Loop Data Labeling Services
Integrate Human-in-the-Loop (HITL) systems into your AI model training with Sapien's expert data labeling services. Our extensive network of over 350 full-time and 30,000 part-time labelers delivers high-quality labels for data across multiple applications, including large language models, computer vision, and document parsing.
The quality control process in Sapien's HITL system ensures superior AI model training by combining automated checks with human oversight. This process captures detailed tagger metrics, which feed into heuristic and lint rules to detect inaccuracies and quality issues. Human experts conduct spot checks, correcting errors and reintroducing them as "red rabbits" to further refine the system. This dynamic interaction creates a feedback loop that continuously improves data quality.
Improve your AI's accuracy, transparency, and reliability with our proven HITL approach. Contact us today to schedule a consult and learn how our data labeling solutions can improve your AI projects.