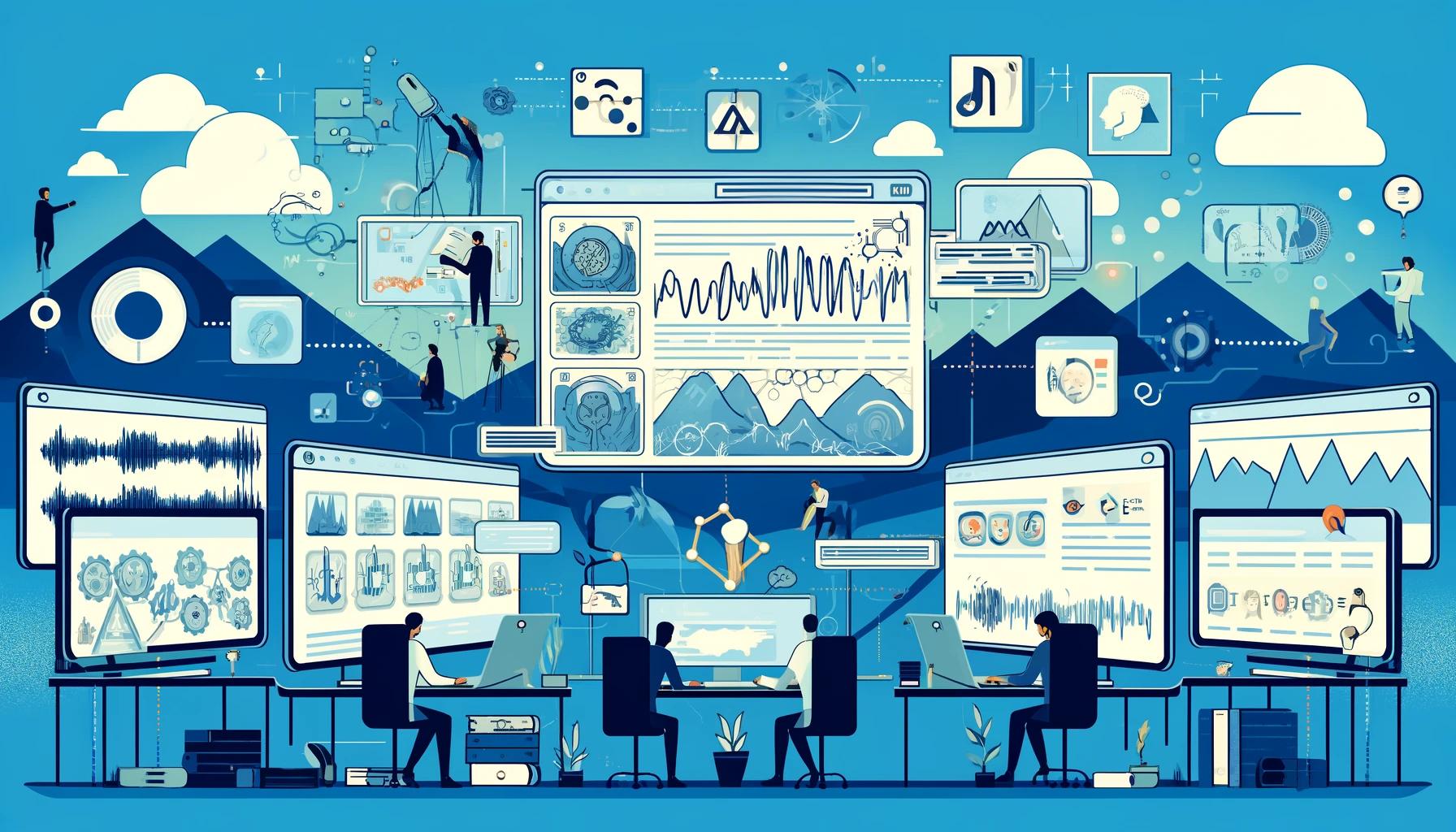
In the artificial intelligence (AI) and machine learning (ML) industries, data labeling and annotation services are a critical component driving innovation and advancement. As businesses and organizations across various industries strive to start using AI and ML, the demand for high-quality, accurately labeled datasets has skyrocketed. Let’s review why data labeling and annotation services are so important, exploring their significance, techniques, challenges, and some best practices.
The Importance of Data Labeling and Annotation
Data is the lifeblood of AI and ML systems. However, raw data alone is insufficient for training models effectively. Machine learning algorithms require carefully curated, labeled, and annotated datasets to learn patterns, make accurate predictions, and perform complex tasks. This is where data labeling and annotation services help refine the process for their training and deployment.
Data labeling involves assigning meaningful labels or tags to data points, such as images, text, audio, or video, to provide context and enable machine learning models to understand and interpret the data accurately. Annotation, on the other hand, involves adding metadata, such as bounding boxes, keypoints, or segmentation masks, to further enrich the labeled data and provide more granular information for training purposes.
The quality and accuracy of data labeling and annotation directly impact the performance of AI and ML models. Poorly labeled or annotated data can lead to suboptimal results, biased predictions, and even catastrophic failures in real-world applications. Therefore, investing in reliable and efficient data labeling and annotation services is crucial for organizations aiming to build robust and trustworthy AI systems.
Techniques and Tools for Data Labeling and Annotation
In the world of machine learning and AI, accurate data labeling and annotation are crucial for training models and ensuring high performance. These processes encompass a variety of techniques and tools, each tailored to specific data types and use cases. Let's explore some of the most common approaches:
- Image Annotation: Image annotation involves labeling objects, scenes, or regions within an image. Techniques include bounding box annotation, semantic segmentation, instance segmentation, and keypoint annotation. Popular tools for image annotation include LabelIng, VGG Image Annotator (VIA), and CVAT (Computer Vision Annotation Tool).
- Text Annotation: Text annotation focuses on labeling and categorizing text data, such as documents, social media posts, or customer reviews. Common tasks include named entity recognition (NER), sentiment analysis, intent classification, and part-of-speech tagging. Tools like Doccano, Prodigy, and BRAT (Brat Rapid Annotation Tool) are widely used for text annotation.
- Audio Annotation: Audio annotation involves labeling and transcribing speech data, identifying speakers, and marking specific audio events or segments. Tools such as Praat, Audacity, and ELAN (EUDICO Linguistic Annotator) are popular for audio annotation tasks.
- Video Annotation: Video annotation deals with labeling and tracking objects, actions, or events within video frames. It often involves techniques like object detection, object tracking, and action recognition. Tools like VATIC (Video Annotation Tool from Irvine, California), CVAT, and LabelMe are commonly used for video annotation.
- 3D Data Annotation: With the rise of autonomous vehicles, robotics, and augmented reality, 3D data annotation has gained significant importance. It involves labeling and annotating 3D point clouds, meshes, or CAD models. Tools such as CloudCompare, 3D Bounding Box Annotation Tool, and VOTT (Visual Object Tagging Tool) are used for 3D data annotation.
Challenges and Best Practices in Data Labeling and Annotation
While data annotation services are essential for AI and ML development, they come with their own set of challenges and best practices:
- Data Quality and Consistency: Ensuring the quality and consistency of labeled data is crucial. Inconsistent or incorrect labels can lead to poor model performance. Implementing rigorous quality control measures, such as multi-pass annotation, expert review, and inter-annotator agreement, can help mitigate this challenge.
- Data Security and Privacy: Handling sensitive or confidential data during the labeling and annotation process requires strict security and privacy measures. Implementing secure data storage, access controls, and anonymization techniques is essential to protect data integrity and comply with regulatory requirements.
- Scalability and Efficiency: As datasets grow in size and complexity, scaling data labeling and annotation processes becomes a significant challenge. Leveraging automation tools, such as pre-labeling and active learning, can help streamline the annotation workflow and reduce manual effort. Distributed annotation platforms and crowdsourcing can also enable parallel annotation efforts and improve efficiency.
- Domain Expertise: Certain domains, such as healthcare, finance, or legal, require specialized domain knowledge for accurate data labeling and annotation. Collaborating with subject matter experts and providing domain-specific training to annotators can ensure high-quality results in niche areas.
- Continuous Improvement: Data labeling and annotation are iterative processes that require continuous improvement. Regularly monitoring the performance of trained models, collecting user feedback, and updating annotation guidelines can help refine the labeling process and improve overall data quality.
Related Topics and Emerging Trends
Beyond the core aspects of data labeling and crowdsourcing annotation services, several related topics and emerging trends are worth exploring:
- Synthetic Data Generation: Synthetic data generation involves creating artificial datasets that mimic real-world data characteristics. It can help augment existing datasets, address data scarcity issues, and reduce the reliance on manual labeling efforts.
- Federated Learning: Federated learning is a distributed machine learning approach that allows training models on decentralized data without the need for data centralization. It enables collaborative learning while preserving data privacy and security.
- Few-Shot Learning: Few-shot learning focuses on training models with limited labeled examples. It leverages techniques such as meta-learning and transfer learning to enable models to quickly adapt to new tasks or domains with minimal labeled data.
- Active Learning: Active learning is an iterative process that involves selectively labeling data points that are most informative for model training. By prioritizing the labeling of high-impact examples, active learning can reduce the overall labeling effort and improve model performance.
- Explainable AI: Explainable AI aims to provide transparency and interpretability to machine learning models. It involves techniques such as feature importance, counterfactual explanations, and rule extraction to help understand and trust the decisions made by AI systems.
The Advancement of Artificial Intelligence and Machine Learning
Data labeling and annotation services play a pivotal role in the development and advancement of artificial intelligence (AI) and machine learning (ML). By providing high-quality, accurately labeled datasets, these services enable organizations to build powerful and reliable AI systems. Properly labeled data is the backbone of AI, ensuring that algorithms can learn patterns and make accurate predictions.
However, the process of data labeling and annotation is not without challenges. It requires careful consideration of several factors, including data quality, security, scalability, and domain expertise. With the rise of more complex AI models, especially in fields like data labeling for autonomous vehicles, the need for accurate and specialized annotations is greater than ever. For autonomous vehicles, for example, labeled data must be precise and thorough to ensure the systems can recognize and respond to the real world safely and effectively.
As the field of AI continues to evolve, these data labeling services become more important for professionals in the industry. To stay competitive, businesses must not only embrace best practices but also leverage advanced tools and techniques. Solutions such as active learning, semi-supervised learning, and gamification in data labeling can help streamline the process, reduce errors, and speed up the training of models.
By staying at the forefront of innovation in data labeling and annotation, businesses can unlock the full potential of AI, driving forward their AI initiatives and building more efficient and reliable models.
Unlock the Power of Data Labeling and Annotation Services with Sapien
Are you looking to harness the full potential of AI and machine learning for your organization? Sapien's data labeling and annotation services provide the key to unlocking accurate, high-quality training data that empowers your AI models. With our team of over 1M+ contributors worldwide, spanning 235+ languages and dialects, we offer the expertise and flexibility to support your data labeling journey across various industries.
Sapien combines the power of AI and human intelligence to efficiently annotate all input types, from text classification and sentiment analysis to semantic segmentation and image classification. Our human-in-the-loop approach ensures precise data labeling and real-time feedback, enabling you to fine-tune your models for optimal performance. Say goodbye to data labeling bottlenecks and scale your resources quickly with Sapien's efficient labeler management and customizable labeling models.
Don't let data labeling challenges hold you back from achieving your AI goals. Schedule a consult with Sapien today and discover how our data labeling and annotation services can revolutionize your AI projects.