What is Audio Data Collection and Why Is It Important?
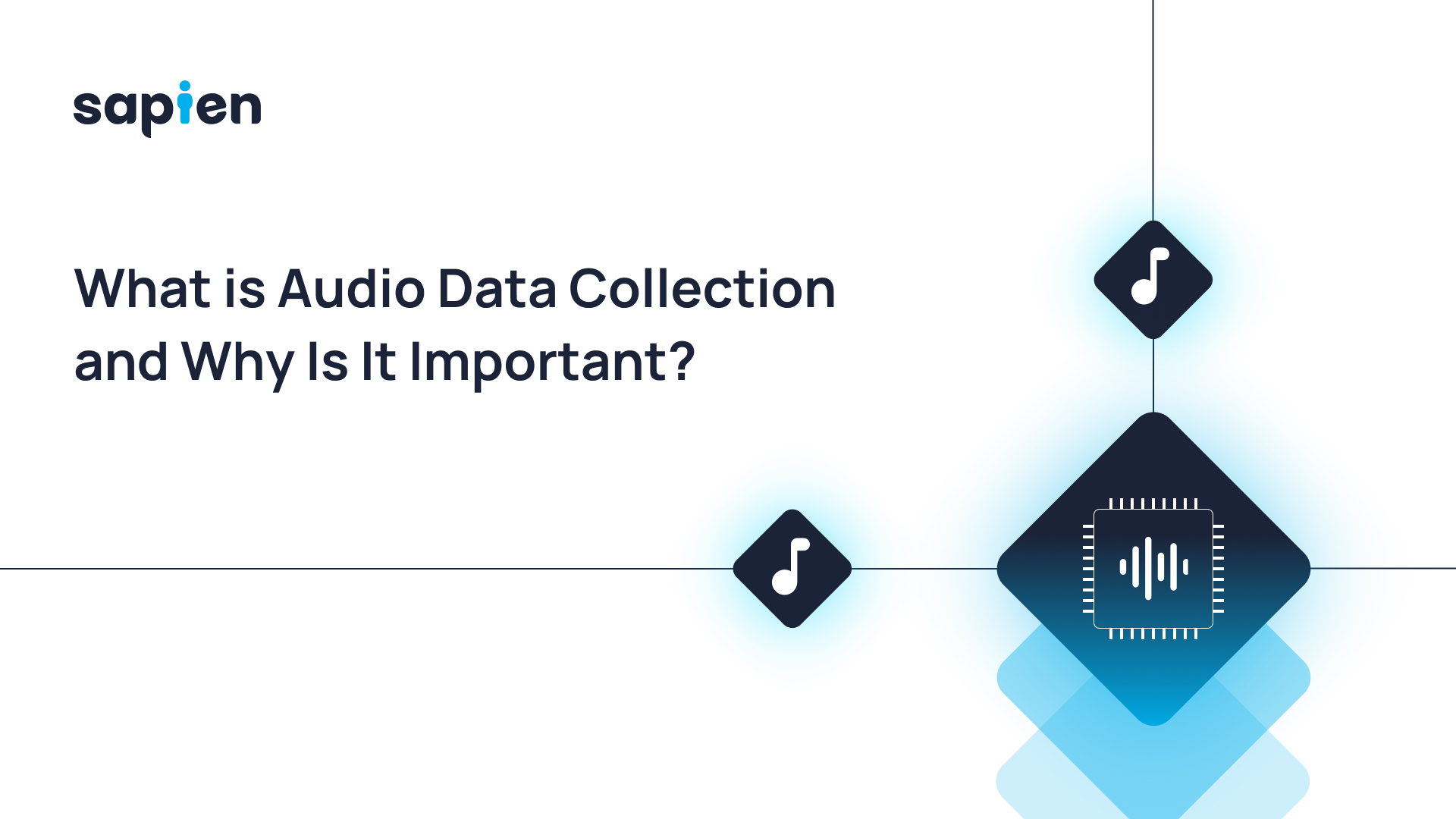
In the artificial intelligence (AI) and machine learning (ML) industries, audio data collection is the first point in a multi-stage process of developing the latest AI models. The ability to collect, process, and analyze audio data enables developers to build voice-activated virtual assistants like Alexa, diagnostic tools in healthcare, and much more. a
Here’s what you should know about audio data collection, and how it is shaping the future of industries around the world with new AI models.
Key Takeaways
- Audio data collection is the process of capturing sound, which may include speech, ambient noise, or sound effects, and preparing it for analysis.
- It is a foundational component for many AI and machine learning models, particularly for tasks like speech recognition and natural language processing (NLP).
- Industries such as healthcare, education, entertainment, and marketing rely on audio data to enhance user experiences, streamline services, and improve data-driven decision-making.
- To ensure high-quality audio data, organizations must choose the right tools, adhere to best practices, and comply with ethical standards.
What is Audio Data Collection?
At its core, audio data collection involves the systematic gathering of audio signals from various sources. These signals can be anything from spoken language to environmental noises, sound effects, or even musical compositions. The primary goal of collecting this data is to extract useful information that can be analyzed, processed, and used to inform machine learning models or applied to different services and products.
Types of Audio Data
There are multiple types of data collection for audio data, each serving different functions depending on the application:
Spoken Language: This form of audio data is used extensively in speech recognition, NLP, and AI voice applications. It involves capturing human speech, which can later be processed and analyzed to understand language patterns, accents, intonations, and more.
Environmental Sounds: Collected from natural or urban environments, these sounds provide context and can improve the realism of AI models used in industries like gaming or virtual reality.
Sound Effects: Artificial or real-world sounds, such as a doorbell or the sound of rain, are often used in audio synthesis, media production, and gaming. These sounds need to be captured with high fidelity for accurate reproduction in digital environments.
Music and Acoustics: High-quality audio data from musical compositions is used in various fields, including entertainment, audio synthesis, and automated music recommendation systems.
Methods of Collecting Audio Data
Audio data collection can be performed through various techniques, depending on the objective and the type of audio being collected. Common audio data collection techniques usually include:
Transcriptions: Transcribing audio data involves converting spoken words into text, either through manual vs automated data collection methods. Automated transcriptions use AI models to convert audio to text in real-time.
Recordings: Audio data can be collected by recording voices or sounds using microphones or specialized recording equipment. This method is widely used in speech recognition and multimedia industries.
Real-Time Audio Capture: This method involves the live capture of audio data, which is often used in surveillance, live streaming, or real-time customer service applications.
In each case, audio data collection requires careful planning and the right equipment to ensure high quality, accuracy, and integrity of the data.
The Importance of Audio Data Collection
Audio data collection is not just a technical process; it is a foundational component that fuels a wide range of modern technologies. Its importance cannot be overstated, especially as industries increasingly rely on AI and machine learning to drive innovation, automate processes, and create more personalized user experiences. The benefits of data collection enable organizations to make informed decisions, enhance predictive capabilities, and tailor services to customer needs. By collecting high-quality audio data, organizations can gain insights that are crucial for decision-making, improving services, and driving operational efficiency.
Enhancing AI and Machine Learning
Audio data plays an integral role in training AI models, particularly in fields like speech recognition, natural language processing (NLP), and sound classification. High-quality, well-annotated audio datasets enable AI systems to learn and interpret complex patterns in human speech, detect emotions, and even identify different speakers in a conversation.
For instance, speech recognition systems like those used by virtual assistants (e.g., Alexa, Siri, and Google Assistant) rely on extensive collections of voice data to accurately transcribe and respond to human commands. This is only possible through the effective collection and annotation of vast amounts of audio data.
Moreover, machine learning models use this audio data to improve speech-to-text conversion, voice authentication, and sentiment analysis. By analyzing variations in pitch, tone, and tempo, these models can even detect specific emotional states or identify unique speakers in multi-speaker environments.
Improving User Experience
The ability to collect and analyze audio data has a profound impact on improving user experiences. Voice-activated services, virtual assistants, and smart home devices are prime examples of how audio data collection is driving personalized and adaptive user experiences.
For instance, voice assistants like Alexa and Google Home use audio data to provide personalized responses based on user preferences. These assistants can adapt their responses based on previous interactions, making the experience more intuitive and user-friendly.
In adaptive learning platforms, audio data is analyzed to provide personalized education experiences for students. Systems can adjust the pace and difficulty of the material based on the learner’s vocal responses or engagement levels, creating a more tailored and effective learning experience.
Data-Driven Decision Making
Audio data is a powerful tool for data-driven decision-making, especially when it comes to gathering insights from customer interactions, market research, and operational processes. By data collection and analyzing audio data from customer feedback or call center interactions, businesses can gain insights into consumer sentiment, detect areas of dissatisfaction, and make informed decisions to improve customer service.
In the healthcare sector, audio data from patient monitoring can help physicians make more informed diagnostic decisions. For instance, analyzing the sound of a patient’s cough, heartbeat, or breathing patterns can provide early indicators of medical conditions, enabling quicker and more accurate diagnoses.
Industries like entertainment and security also use audio data to enhance their decision-making processes. In entertainment, sound engineers rely on audio data to create realistic environments for films, video games, and virtual reality experiences. In security, audio data helps with surveillance and the detection of abnormal sounds, like alarms or breaking glass, to trigger security protocols.
Audio Data Analysis Techniques
Once collected, audio data must undergo various forms of processing and analysis to extract meaningful information. The analysis of audio data involves several techniques, including data analysis tools such as speech recognition, machine learning algorithms, and noise reduction methods.
Speech Recognition: Converting Audio to Text
Speech recognition technology converts spoken language into written text, which can then be analyzed for further processing. This technology underpins numerous modern services, including automated transcription, voice search, and AI-powered virtual assistants.
At a technical level, speech recognition relies on a combination of acoustic models and language models. The acoustic model is trained to recognize the unique sound patterns associated with phonemes (the smallest unit of speech), while the language model is responsible for understanding the context and structure of the spoken language. Together, these models enable the accurate transcription of speech into text, even in noisy environments.
Machine Learning Approaches: Training Models with Audio Data
Machine learning models trained with audio data require robust data annotation and feature extraction processes. These models use techniques like deep learning to learn from large datasets of annotated audio signals.
For example, in supervised learning, a machine learning model is trained using labeled audio data, where the correct output is known. This method helps the model learn to classify or predict outcomes based on new audio data. Unsupervised learning approaches, such as clustering or dimensionality reduction, are also used to uncover patterns in audio data without predefined labels.
The power of machine learning in audio data processing is particularly evident in applications like emotion detection, speaker identification, and audio classification.
Noise Reduction Techniques: Enhancing Audio Quality
The quality of the collected audio data is critical for accurate analysis, and noise reduction techniques play a pivotal role in improving the clarity of the data. Spectral subtraction, adaptive filtering, and beamforming are common noise reduction techniques used to minimize background noise and isolate the desired audio signal.
In spectral subtraction, for example, the algorithm estimates the noise in the audio signal by identifying frequency components that do not vary significantly over time. These frequencies are then subtracted from the signal, leaving behind the cleaner, desired audio.
Noise reduction is especially important in applications like telemedicine, where the clarity of a patient's voice or heartbeat can significantly affect diagnostic outcomes.
Audio Feature Extraction: Key Attributes for Analysis
To analyze audio data effectively, it is essential to extract key features from the raw audio signal. Audio feature extraction involves identifying attributes such as pitch, frequency, tempo, and spectral content, which are then used in machine learning models to classify or predict outcomes.
Common feature extraction techniques include Mel-Frequency Cepstral Coefficients (MFCCs), Chroma feature extraction, and zero-crossing rate analysis. These features help in applications such as speech recognition, audio classification, and music information retrieval.
Audio Data Collection in Different Industries
The applications of audio data collection are not limited to tech-driven industries; they extend to virtually every sector, including healthcare, education, entertainment, and marketing. Each of these industries relies on audio data to improve efficiency, provide better services, and enhance user experiences.
Healthcare: Improving Patient Care and Diagnostics
In the healthcare industry, audio data collection is critical for telemedicine and remote patient monitoring. Audio signals such as coughs, heartbeats, and breathing patterns can be captured and analyzed to provide insights into a patient's health condition.
For example, AI models trained on audio data can identify respiratory issues by analyzing the sound of a patient’s breathing. Voice biomarkers are another area of exploration, where audio data is used to detect conditions like Parkinson’s disease, dementia, or depression based on changes in speech patterns.
Education: Facilitating Learning and Engagement
In education, audio data is being used to create adaptive learning platforms that cater to individual learning styles. By collecting and analyzing voice data from students, these platforms can adjust the pace, difficulty, and content of the material to match each learner’s needs.
For remote education, audio data is used for automated grading of spoken assignments, speech assessments, and real-time feedback during lessons. This helps teachers provide more personalized feedback and improves the overall learning experience.
Entertainment: Enriching Content Creation and User Experience
The entertainment industry uses audio data in virtually every facet of content creation. From sound mixing in films to creating realistic soundscapes in video games, high-quality audio data is essential for creating immersive experiences.
Gaming companies collect environmental sounds and voice recordings to create more immersive gaming environments. Music streaming services also rely on audio data to power their recommendation algorithms, ensuring that users are presented with music that aligns with their tastes and preferences.
Marketing and Advertising: Driving Consumer Insights
In marketing, audio data provides valuable insights into consumer behavior and sentiment analysis. Brands can collect audio data from customer interactions in call centers or voice-activated devices to better understand consumer preferences and improve their advertising strategies.
By analyzing voice data, companies can create more personalized marketing campaigns, identify customer pain points, and refine product offerings.
Best Practices for Audio Data Collection
To collect high-quality and actionable audio data, it is essential to follow best practices that ensure the accuracy, integrity, and ethical handling of the data.
Choosing the Right Tools
The success of any audio data collection project hinges on the choice of audio data collection tools. Tools like high-quality microphones, audio recording software, and cloud-based data storage platforms are critical for gathering, storing, and processing audio data. Microphone placement and environmental control also play a significant role in ensuring the quality of the recorded audio.
Ensuring Quality and Accuracy
Achieving high-quality audio data requires a controlled environment with minimal background noise. Choosing the right microphone for the task, using soundproof rooms, and employing post-processing techniques like noise reduction are essential steps in ensuring data quality. Clear, high-quality audio ensures that the subsequent analysis or machine learning training yields accurate and reliable results.
Compliance and Ethical Standards
In any audio data collection project, adhering to ethical standards and ensuring compliance with privacy laws is crucial. This is especially important in industries like healthcare and marketing, where the misuse of audio data could result in privacy violations. Obtaining explicit consent from participants and anonymizing sensitive data are essential practices that ensure the ethical use of audio data.
Get Audio Data Collection to Support Your AI Model Development with Sapien
At Sapien, we build customized audio data collection pipelines tailored to your specific project needs. With a focus on quality, accuracy, and ethical standards, we ensure that the audio data we collect is ready for high-level analysis and training.
Whether you are looking to improve your speech recognition models, enhance user experiences, or gain deeper consumer insights, Sapien has the expertise and tools to drive your project forward.
Unlock the full potential of audio data collection with Sapien. Schedule a consult to discuss your project needs, and let us design a custom pipeline that ensures the best possible outcomes for your AI initiatives.
FAQs
Who can benefit from Sapien’s audio data collection?
Organizations in AI development, healthcare, education, entertainment, and marketing can all benefit from Sapien’s tailored audio data collection solutions.
How do I get started with an audio data project using Sapien?
Contact our team to schedule a consultation. We’ll guide you through the process of designing and implementing a custom audio data collection pipeline that meets your project’s needs.
How can audio information be collected and compared?
Audio information can be collected using a variety of methods such as recordings, real-time capture, and transcriptions. Once collected, machine learning algorithms can be used to compare and analyze the data for insights.