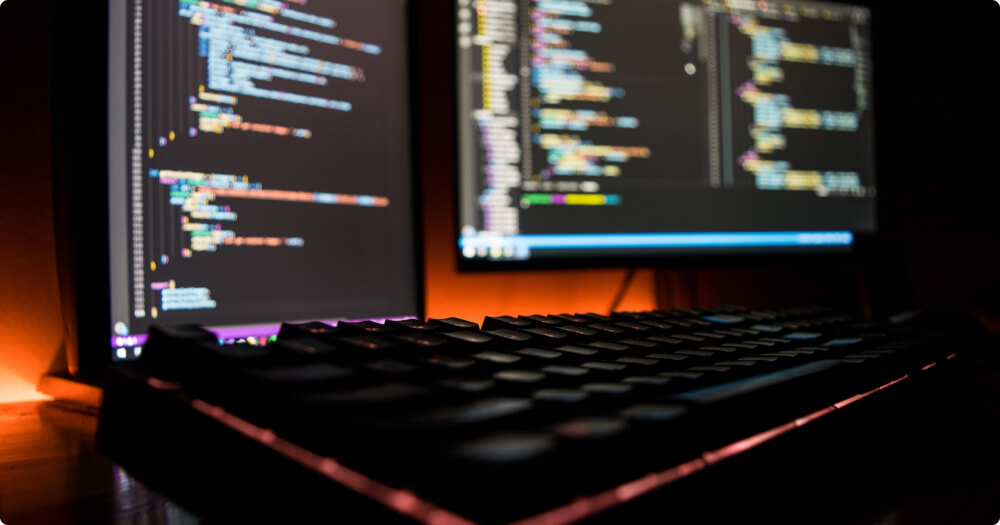
Data labeling is often thought of as the less glamorous side of machine learning and artificial intelligence. While it may not make headlines like the latest advancements in AI, it's a fundamental step in the process of training AI models, especially in the realm of supervised learning. The process might seem straightforward—assign labels to data points so that a machine learning model can learn from them. However, the reality is far more complex and involves considerable time, effort, and financial resources.
Time-Consuming Nature of Data Labeling
When it comes to developing a machine learning project, data labeling is not a quick step you can breeze through. It's surprising to many that data preparation, including labeling, takes up almost 80% of the entire project time. Whether you're working on image recognition or natural language processing, each data point often needs to be meticulously labeled by human experts to ensure accuracy. And if your project has millions of these data points, the time factor becomes an increasingly heavy burden. For instance, in healthcare AI projects that require labeling medical images, delays in data labeling can significantly extend project timelines and even impact patient care.
Labor-Intensive Aspect
Besides being a drain on time, data labeling is also labor-intensive. The reason is simple: quality labeling requires human judgment. While there are tools and software that can assist in the labeling process, the final check usually needs a human touch. This becomes even more pronounced in specialized sectors like healthcare, where the labelers need to have a certain level of expertise to accurately label data such as medical images or patient records. The need for specialized skills adds another layer of complexity and cost to the process.
Financial Cost
Speaking of cost, the monetary aspect of data labeling is something that can't be overlooked. The costs add up quickly when you factor in the human resources needed for a large-scale labeling project. Whether you're hiring in-house experts or outsourcing to specialized labeling services, each comes with its own price tag. For smaller organizations or projects with limited budgets, these costs can be a significant barrier, restricting their ability to leverage AI to its fullest extent. While data labeling is a critical step in machine learning development, its challenges are often underestimated. The process is far from simple, involving significant investments of time, human resources, and money. Although some of these challenges can be mitigated through automated labeling methods or crowd-sourcing, the fundamental issues still remain. As the field of AI continues to grow, understanding and addressing the intricacies of data labeling will be essential for developing robust and reliable AI models.
Get in Touch with Sapien to Book a Demo and See How We Solve the Biggest Challenges of Data Labeling Through Our Consumer Game
At Sapien, we recognize the complexities and challenges involved in data labeling. That's why we've developed a unique, gamified approach to data preparation for AI training. Our method not only ensures data quality but also addresses the scalability issue, allowing you to handle large datasets without compromising accuracy. And the best part? Our approach significantly reduces the costs associated with data labeling. So why struggle with the bottlenecks of traditional data labeling methods when you can streamline the process with Sapien? Book a demo with us to see how we can help you tackle the biggest challenges in data labeling effectively.