LLM Hallucinations and the Threat to Your AI Models
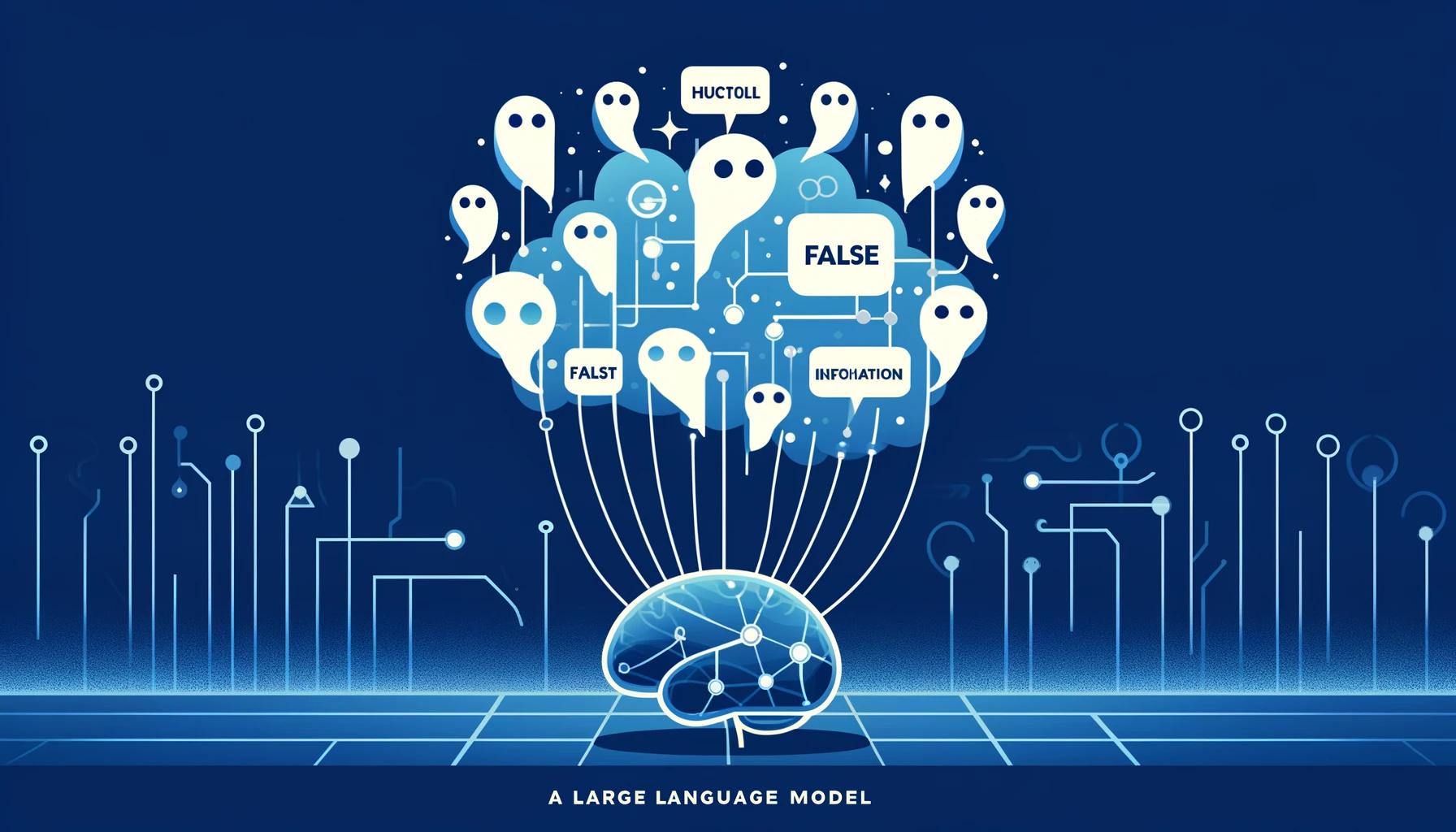
Hallucinations are concerning occurrences that can happen when an artificial intelligence (AI) system like a large language model (LLM) generates information that appears factual and truthful on the surface, yet has no actual basis in reality or alignment with the context originally provided to the AI model. These false statements can seem quite convincing to readers at first glance, making it crucial that we enhance our understanding of the root causes of such AI hallucinations. It is important that we explore effective mitigation strategies to address the risks posed by AI systems generating fabricated claims that lack factual verification, and how data labeling services can help mitigate the worst effects of LLM hallucinations.
What Causes LLM Hallucinations?
Several key factors can contribute to an LLM beginning to hallucinate false information that mimics truthful facts and details. One element is pure overconfidence in the model's ability to continue generating coherent text, despite having no actual knowledge or true semantic understanding of the topic at hand. The model essentially keeps writing sentences that sound plausible in succession, whilst failing to ever verify the objective accuracy of the claims being made.
A second factor is the model's relentless attempt to put together reasonable-sounding narratives that flow logically from sentence to sentence, whilst neglecting to check whether the core claims align with verifiable facts. Here, the model becomes overly focused on textual coherence rather than factual integrity. Next, we cannot ignore the insufficient techniques applied during the initial training process, which fail to properly distinguish factual statements based in reality from complete fiction.
Without the right training, the model struggles to differentiate real factual knowledge from fabricated falsehoods. On top of all of this, substantial bias in the datasets used for pre-training models can also contaminate the generation process. The lurking distortions and societal biases ingrained in the training data are absorbed by the model and then reflected in its outputs.
Types of LLM Hallucinations
Looking closer, we can identify distinct categories of false information commonly seen as a result of LLM hallucinations. One type is the generation of wholly convincing but utterly fictitious "false facts" - statements confidently presented as factual truth when in reality no basis for the claim exists. A second flavor manifests as elaborate and outwardly coherent "fantastical narratives" - stories woven start to finish by the model that flow logically yet have zero factual validity when examined against actual events in the real world.
Occasionally, anachronisms emerge, with the model situating people, places, events or ideas in incorrect or impossible historical time periods that do not align with timelines of when the elements existed in reality. We also see frequent "attribution errors" where the model ascribes specific quotes or ideas to the wrong sources, rather than crediting their genuine originators. This can make information unusable and in some cases dangerous to the end user.
Technical Explanations for LLM Hallucinations
When we analyze hallucinations on a technical level, certain architectural limitations of large language models clearly contribute to these false information generation issues:
Most models struggle fundamentally to link the claims they generate back to verification through any form of real-world knowledge or grounded reasoning that could validate the statements as facts. This lack of commonsense reasoning makes it almost impossible to confirm whether generated claims have a basis in reality.
Secondly, there appears to be intrinsic difficulty constraining the models' production of logically coherent but completely unverified textual content. The prevailing model architectures crafted primarily for fluency and coherence can far too easily produce fictional written content that sounds highly plausible on first pass.
Of course, most models fail to include robust discernment to differentiate whether their generated statements align with patterns observed in training datasets versus false inferences invented entirely by the model itself. In essence, the models often struggle to distinguish correlations learned from actual data versus fabrications of their own creation.
Risks and Concerns Around LLM Hallucinations
When advanced AI systems hallucinate fictional details or entirely false information as facts, it poses major risks that cannot be ignored:
Primarily, there is the potential spread of dangerous misinformation if the hallucinated content is exposed publicly without thorough verification first. The AI’s false claims risk being accepted as truth by readers.
Societal biases could also become implicitly reinforced if the models indirectly mimic distorted trends absorbed from biased training datasets. This could propagate harmful biases rather than correcting them.
As advanced AI is increasingly trusted for authoritative knowledge on diverse topics, hallucinations erode public trust in the integrity of the systems. Once deemed unreliable, it becomes hard to restore confidence.
Mitigation Strategies
In response to the pressing issue of dangerous AI hallucinations, researchers are actively exploring mitigation strategies, such as:
Improved training techniques focused specifically on teaching models to generate verifiable factual statements rather than unverified claims across a diverse range of topics. Hallucinations must be minimized from the start of model development.
With this, diligent efforts to proactively identify model blind spots around sensitive subject areas prone to hallucinations so corrective interventions can be made on those weaknesses.
Thirdly, adding constraints during text generation to force models to source any factual statements to credible references rather than hallucinating facts.
Finally, clearly disclaiming any speculative content without factual verification generated by models to caution users on potentially false information.
Real-World Impacts of Unchecked LLM Hallucinations
Allowing problematic hallucinations in LLMs poses significant risks not just theoretically but in practice as the systems are deployed in the real world. Unverified false claims spread by overconfident LLMs could negatively impact:
- Public discourse if hallucinated misinformation affects political, social, or scientific discussions.
- Personal lives if incorrect medical, financial or other private information is generated or shared.
- Organizational decisions if models inform choices with faulty data.
- Legal liability if harm comes from actions based on an LLM's hallucinated guidance.
Public Perception Around LLM Hallucinations
It is important that public perception of this issue balances an appreciation for tremendous good enabled by helpful LLMs with vigilance against unchecked harms from potential hallucinations:
- The public should be made aware of risks as LLMs become more prevalent.
- However, this should not overshadow the many benefits of LLMs when responsibly deployed.
- Realistic understanding can compel increased accountability from those developing and deploying LLMs.
Building User Trust Through Responsible LLM Usage
Responsible disclosure and usage practices by those deploying LLMs can help build appropriate public trust:
- Clearly convey limitations and have human oversight over hallucinated content.
- Develop efficient workflows for reporting and correcting errors.
- Leverage selective disambiguation to prompt LLMs to verify uncertain statements.
A Concerning but Manageable Limitation
The generation of seemingly factual but objectively false information by AI systems presents concerning limitations as large language models become more capable and widely deployed. Through focused mitigation strategies rooted in better training, constraints, responsible use and disclosure around actual model capabilities, the AI research community must continue making progress to curtail risks posed by AI hallucinations.
There remains a lot of work to ensure these powerful systems emphasize generating actual truth over fabricated claims that merely sound plausible. Researchers should continue working diligently so that the public can enjoy the benefits of advanced language models without the dangers of AI hallucinations.
Mitigating LLM Hallucinations Through Responsible Human Oversight with Data Labeling by Sapien
While progress continues on technical strategies to address AI hallucinations, responsible human oversight remains essential for catching false information before it spreads. Companies like Sapien enable this through our combination of global human expertise and AI technology for data labeling.
Sapien's network of human domain experts across fields like law, medicine, and engineering ensures highly accurate analysis of complex data that LLMs may misunderstand or hallucinate false details about. Our technology platform also facilitates efficient workflows for real-time human validation of any unsure LLM outputs before public exposure.
This human-AI symbiosis allows consumers and organizations to benefit from advanced language models while safeguarding against potentially inaccurate outputs through verification by true subject matter experts. As long as humans remain in the loop to provide quality control on high-stakes LLM output, we can continue driving AI progress to assist humanity while proactively addressing the risks of unchecked hallucinations.
Contact Sapien today to learn more about our data labeling services to mitigate the risks of LLM hallucinations and book a demo to experience our platform.