In Conversation: Sapien COO Henry Chen on the Future of Data Labeling, Automation, and Scaling for AI
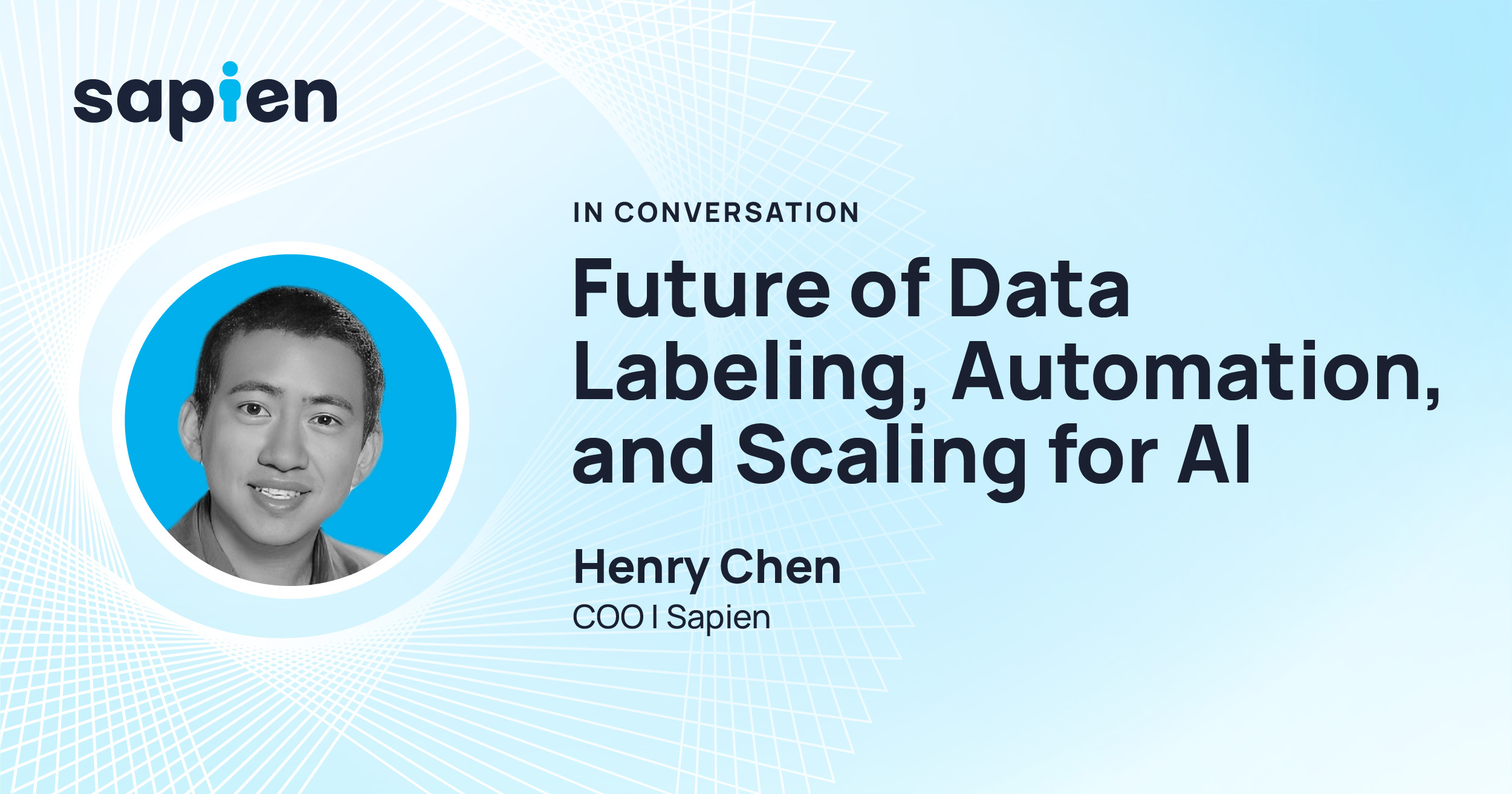
As AI models become more capable and use cases expand, data labeling has become even more important for building accurate and reliable models. We sat down with Sapien COO Henry Chen, to discuss the current state of data labeling and how it’s evolving, and insights into how Sapien is adapting to meet the demands of the AI industry.
1. How do you see the role of data labeling evolving in the age of AI? Do you anticipate a shift towards more automated processes, or will human expertise remain crucial?
I think data labeling’s role is definitely evolving as AI grows, and we’re already seeing that with more automated tools becoming a part of the process. At Sapien, we’re big on automation as a way to get data ready for annotation faster and more efficiently, but human expertise is still a huge part of the picture. Ground truth data—basically the most accurate data—is still what we need to make AI models that actually work well in the real world. So, while automation will speed up and streamline parts of data preparation, human insight and accuracy will continue to be crucial in creating the best data for training AI. As the demand for AI expands, I believe that we’ll need more people with specialized skills, not fewer, to meet the growing needs of the industry.
2. What are the biggest challenges you foresee in scaling data labeling operations to support the growing demand for AI models? How are we addressing these challenges at Sapien?
Scaling data labeling operations to support AI’s rapid growth has its challenges, especially with the sheer amount of ground truth data that’s needed. More companies are developing AI models, and all of them require clean, labeled data to train on, so the demand is skyrocketing. One of the ways we’re addressing this at Sapien is by building a decentralized network of labelers. This is going to be essential as we move forward because a centralized labeling facility can only do so much. While these facilities work well for now, I don’t see them being a scalable solution for the future. By decentralizing, we’re not only able to meet larger demands but also keep up with fluctuating needs around the globe, making us more adaptable to whatever challenges lie ahead.
3. How do you ensure the quality and consistency of our data labeling output, especially as we work with increasingly complex datasets?
Quality and consistency are non-negotiables, especially as the datasets we work with get more complex. Ensuring our output remains top-notch takes a lot of experience and oversight. Our focus on quality control means we can take on more challenging projects while still delivering data that our clients can count on.
4. What are your thoughts on the future of AI model development and deployment? How do you see Sapien contributing to these advancements?
In terms of AI model development and deployment, I see Sapien as a critical part of moving AI forward. Right now, there’s tons of focus on building better compute power and more efficient algorithms—big companies and brilliant minds are all over that. But data annotation, on the other hand, is still kind of stuck in traditional ways of doing things, and that’s holding back the whole field. Sapien’s mission is to change that by bringing in fresh solutions and a new approach to data labeling. We want to eliminate this bottleneck so AI can progress faster and be more impactful in everyday applications.
5. How are we staying ahead of the curve in terms of emerging technologies and trends in the data labeling industry? What investments are we making to ensure our continued success?
We’re putting a lot of resources into developing the best data flow processes possible. We’re also focusing heavily on 3D and 4D data types because we know that’s where the industry is headed. These kinds of data open up huge possibilities for training more advanced AI models, and by investing now, we’re positioning ourselves to meet future demands. This forward-looking approach is about making sure Sapien stays not just relevant but a leader in the data labeling industry, capable of delivering exactly what’s needed as AI continues to evolve.
As Henry highlighted, Sapien’s commitment to quality, adaptability, and foresight is helping the company meet the demands of an industry constantly pushing forward. With a balanced approach that embraces both automation and human expertise, Sapien is setting the standard for whatever comes next for AI.