Data Labeling for AI: A Gateway to Advanced Machine Learning
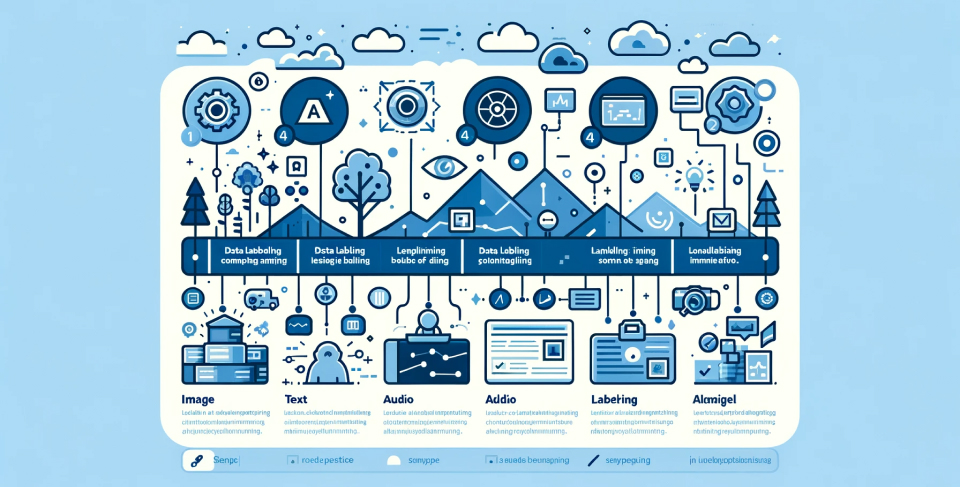
For artificial intelligence (AI), data labeling is a fundamental and indispensable process. It's the meticulous task of annotating and categorizing raw data to make it comprehensible and usable for machine learning models. Let's review some of the key components of data labeling for AI, exploring its methodologies, significance, and future trajectories. Here's how high-quality data labeling acts as a catalyst in the advancement of machine learning technologies.
What is Data Labeling in Machine Learning?
Data labeling is the cornerstone of machine learning, a subset of AI. It involves annotating raw data whether it's images, text, or audio with relevant labels that define and categorize the information contained within. By tagging data with accurate labels, machine learning algorithms can interpret and learn from it effectively. With the advent of data labeling tools, the process has become more efficient, enabling faster model training and better accuracy in AI predictions.
Types of Data Labeling:
Data labeling is a crucial component of machine learning, providing the foundation for AI models to learn and make predictions based on structured data. There are several types of data labeling methods, each tailored to different kinds of data and specific AI applications. Understanding these methodologies is essential to ensure the accuracy and efficiency of the labeled data used to train AI systems.
Whether it’s text classification, image annotation, audio transcription, or video annotation, each method plays a significant role in making raw, unstructured data usable for machine learning algorithms. Here, we explore some of the most commonly used data labeling techniques.
Image Annotation
Image annotation involves labeling visual elements within an image to make them understandable by AI systems. This technique is critical in many AI-driven applications, especially those involving computer vision.
- Purpose of Image Annotation: Involves labeling visual elements within an image to identify objects, features, or boundaries.
- Key Applications: Essential for computer vision, autonomous vehicles, facial recognition, and medical imaging.
- Autonomous Driving Example: Labeled images help AI systems recognize pedestrians, traffic signs, and vehicles, ensuring safe navigation.
- Types of Image Annotation:some text
- Object Detection: Labeling specific objects (e.g., cars, people) with bounding boxes.
- Image Segmentation: Dividing images into regions corresponding to different objects or backgrounds.
- Impact: Helps AI models recognize visual patterns and is essential in various AI applications.
Text Categorization
Text classification is a fundamental process in Natural Language Processing (NLP) that involves categorizing text data into predefined tags or categories. This allows AI models to better understand the context, sentiment, and intent of text.
- Purpose of Text Classification: Categorizing text data into predefined groups or tags to help AI understand the context and meaning.
- Applications in NLP:some text
- Sentiment Analysis: Identifying emotional tone (positive, negative, neutral).
- Topic Classification: Labeling text based on subjects such as politics, sports, or technology.
- Entity Extraction: Extracting specific information like names, dates, and locations.
- Use Cases: Crucial for chatbot training, information retrieval, and customer service automation.
- Impact: Ensures high accuracy in interpreting human language, making it foundational in NLP.
Audio Transcription
Audio transcription is the process of converting spoken language into written text, allowing AI systems to process and respond to verbal inputs. It plays a significant role in applications like speech recognition and voice assistants.
- Purpose of Audio Transcription: Converts spoken language in audio files into written text for AI models to interpret.
- Applications: Used in speech recognition, voice assistants, and customer service systems.
- Transcription Process: Involves labeling audio with text and adding annotations like speaker identification and emotion detection.
- Real-World Examples: Helps virtual assistants like Siri and Alexa, transcription services, and real-time translation tools.
- Impact: Proper labeling improves the AI’s ability to understand and respond to verbal inputs, enhancing user experience.
Video Annotation
Video annotation involves tagging objects, actions, or events in video sequences. This technique is essential for AI systems that need to analyze dynamic, real-time visual data, such as in autonomous driving or sports analytics.
- Purpose of Video Annotation: Involves identifying and labeling objects, actions, or events within video sequences.
- Key Applications:some text
- Used in video surveillance, autonomous vehicles, and sports analytics.
- Critical for training AI systems to recognize and track moving objects, like pedestrians or vehicles, in real-time.
- Types of Video Annotation:some text
- Action Recognition: Labeling specific activities or movements.
- Object Detection: Identifying and tracking specific items or people.
- Event Detection: Labeling sequences that represent significant events (e.g., accidents, sports goals).
- Impact: AI models learn to predict future events or movements, vital for systems relying on real-time visual inputs.
Enhancing AI Models with Quality Data Labeling
High-quality data labeling for AI directly impacts the performance of AI models. When machine learning models are trained with accurate, diverse, and representative labeled data, they become more effective in performing tasks and making predictions.
The Importance of Accurate Data Labeling for AI Success
Accurate data annotation plays a critical role in the success of AI and machine learning systems. It involves annotating raw data with the correct labels to make it usable for training algorithms. Below are key reasons why accurate data labeling is essential for AI success:
- Precision and Accuracy: Labels must be exact and consistently applied to ensure the AI model's performance.
- Diversity in Data: A wide range of labeled data helps in building robust models that perform well across various scenarios.
- Mitigating Bias: Careful and unbiased labeling is critical to prevent AI models from developing skewed perspectives.
How Consistent Data Labeling Improves AI Model Performance
Consistency in data labeling is crucial for improving AI model performance. When data is labeled consistently, AI systems can reliably learn and make accurate predictions. Inconsistent labeling introduces confusion, leading to errors and poor model performance.
For example, in sentiment analysis, inconsistent labels like marking positive sentiment as neutral can confuse the model. Similarly, in image annotation, labeling a cat as "animal" instead of "feline" can cause misclassification. Consistent labeling ensures clear, reliable data, allowing the model to generalize better to new data, enhancing accuracy.
The Role of Professional Data Labeling Services
Given the complexity and scale of data labeling, professional services play a vital role. These services offer expertise in accurately labeling large datasets, ensuring data security, and providing scalable solutions to meet the demands of AI projects. Here are the advantages of professional data labeling:
- Expertise and Experience: Professional labelers bring a deep understanding of the nuances involved in data labeling.
- Scalability: Ability to handle vast amounts of data efficiently.
- Quality Assurance: Ensuring high standards of accuracy and consistency.
- Data Security: Adherence to strict protocols to protect sensitive data.
Future of Data Labeling in AI
The landscape of data labeling is evolving rapidly, driven by technological advancements and the ever-increasing demands of AI development. The future of data labeling is evolving with advancements in automated data labeling. Here are some upcoming trends and innovations:
- Automated Labeling Technologies: The integration of AI in the labeling process to automate and expedite the task.
- Active Learning Strategies: Where AI models identify and prioritize data that would be most beneficial for labeling.
- Crowdsourced Labeling: Leveraging a distributed workforce to annotate data, enhancing scalability and diversity.
- Advanced Annotation Tools: Development of sophisticated tools offering enhanced precision and efficiency in labeling tasks.
Upgrade Your AI Projects with Sapien's Expert Data Labeling Solutions
Data labeling for AI is a critical process that lays the foundation for the success of machine learning models. The quality, accuracy, and comprehensiveness of data labeling determine the effectiveness of AI applications across various fields. As we move forward, the importance of professional data labeling services, equipped with cutting-edge tools and methodologies, will only grow.
If you're looking to improve or develop AI and require high-quality data labeling services, Sapien is your partner. Offering a blend of expertise, advanced technology, and scalability, Sapien stands at the forefront of data labeling services.
Schedule a Consult with Sapien today and revolutionize your AI projects with unparalleled data labeling solutions.